Latent profile analysis identified COVID-19 ARDS phenotypes in Thai patients: Research protocol and preliminary report
The phenotype of SARS-CoV-2 respiratory failure in Thai patients
DOI:
https://doi.org/10.54205/ccc.v30.256078Keywords:
Phenotype, SARS-CoV-2, COVID-19, Acute respiratory distress syndromeAbstract
Background: Clinical heterogeneity was observed among COVID-19 patients with acute respiratory distress syndrome (CARDS). The heterogeneity of disease was contributed to different clinical progression, responses to treatment, and mortality.
Objective: We aim to study the phenotype and associated mortality of COVID-19 respiratory failure in Thai patients.
Methods: We conducted a single-center, retrospective observational study. The data were collected in CARDS who received an invasive mechanical ventilator in ICU. Patient-related data were collected at admission before the onset of respiratory failure. The main features include demographics data, SOFA score, laboratory, CXR severity score, treatment during hospitalization, and the following data at the onset of respiratory failure during invasive mechanical ventilator. We also collected patients’ status at 28-day, in-hospital complications, and ventilator-free days at 28-day after intubation. The latent profile analysis was performed to identify distinct phenotypes. After identifying phenotypes, characteristics and clinical outcomes were compared between phenotypes. The primary outcome was the phenotype and associated mortality of COVID-19 respiratory. Secondary outcomes include characteristics of phenotype, ventilator-free days, response to treatment, and complications in each phenotype.
Discussion: This study aims to identify the phenotype of COVID-19 Respiratory Failure in Thai Patients The different phenotypes may be associated with varying responses to treatment and outcomes that the result of this study may be useful for determining treatment and predicted prognosis of COVID-19 Respiratory Failure In Thai Patients.
Ethics and dissemination: The study protocol was approved by the Institution Review Board of Ramathibodi Hospital, Mahidol University, Thailand (No. MURA2021/740). We plan to disseminate the results in peer-reviewed critical care medicine or pulmonology related journal, conferences nationally and internationally.
References
Tzotzos SJ, Fischer B, Fischer H, Zeitlinger M. Incidence of ARDS and outcomes in hospitalized patients with COVID-19: A global literature survey. Critical Care. 2020;24(1).
RECOVERY Collaborative Group, Horby P, Lim WS, Emberson JR, Mafham M, Bell JL, et al. Dexamethasone in Hospitalized Patients with Covid-19. N Engl J Med. 2021;384(8):693-704.
Rosas IO, Bräu N, Waters M, Go RC, Hunter BD, et al. Tocilizumab in Hospitalized Patients with Severe Covid-19 Pneumonia. N Engl J Med. 2021;384(16):1503-1516.
Calfee CS, Delucchi K, Parsons PE, Thompson BT, Ware LB, et al. Subphenotypes in acute respiratory distress syndrome: latent class analysis of data from two randomised controlled trials. Lancet Respir Med. 2014;2(8):611-20.
Calfee CS, Delucchi KL, Sinha P, Matthay MA, Hackett J, et al. Acute respiratory distress syndrome subphenotypes and differential response to simvastatin: secondary analysis of a randomised controlled trial. Lancet Respir Med. 2018;6(9):691-698.
Famous KR, Delucchi K, Ware LB, Kangelaris KN, Liu KD, et al. Acute Respiratory Distress Syndrome Subphenotypes Respond Differently to Randomized Fluid Management Strategy. Am J Respir Crit Care Med. 2017;195(3):331-338.
Sinha P, Calfee CS, Cherian S, Brealey D, Cutler S, et al. Prevalence of phenotypes of acute respiratory distress syndrome in critically ill patients with COVID-19: a prospective observational study. Lancet Respir Med. 2020;8(12):1209-1218.
Ranjeva S, Pinciroli R, Hodell E, Mueller A, Hardin CC, Thompson BT, et al. Identifying clinical and biochemical phenotypes in acute respiratory distress syndrome secondary to coronavirus disease-2019. EClinicalMedicine. 2021;34(100829):100829.
A. Abo-Hedibah S, Tharwat N, H. Elmokadem A. Is chest X-ray severity scoring for covid-19 pneumonia reliable? Polish Journal of Radiology. 2021;86(1):432–9.
Oberski D. Mixture models: Latent profile and latent class analysis. In: Human–Computer Interaction Series. Cham: Springer International Publishing; 2016. p. 275–87
Mahat RK, Panda S, Rathore V, Swain S, Yadav L, Sah SP. The dynamics of inflammatory markers in coronavirus disease-2019 (COVID-19) patients: A systematic review and meta-analysis. Clin Epidemiol Glob Health. 2021;11(100727):100727.
Antunez Muiños PJ, López Otero D, Amat-Santos IJ, López País J, Aparisi A, Cacho Antonio CE, et al. The COVID-19 lab score: an accurate dynamic tool to predict in-hospital outcomes in COVID-19 patients. Sci Rep. 2021;11(1):9361.
Karimi A, Shobeiri P, Kulasinghe A, Rezaei N. Novel systemic inflammation markers to predict COVID-19 prognosis. Front Immunol. 2021;12:741061.
Hariyanto TI, Japar KV, Kwenandar F, Damay V, Siregar JI, Lugito NPH, et al. Inflammatory and hematologic markers as predictors of severe outcomes in COVID-19 infection: A systematic review and meta-analysis. Am J Emerg Med. 2021;41:110–9.
Parimoo A, Biswas A, Baitha U, Gupta G, Pandey S, Ranjan P, et al. Dynamics of inflammatory markers in predicting mortality in COVID-19. Cureus. 2021;13(10): e19080.
Dolnicar S, Grün B, Leisch F, Schmidt K. Required sample sizes for data-driven market segmentation analyses in tourism. Journal of Travel Research. 2014;53(3):296-306.
Pinheiro, J.C. and Bates, D.M., Approximations to the log-likelihood function in the nonlinear mixed-effects model. Journal of computational and Graphical Statistics. 1995;4(1), pp.12-35
Banfield, J.D. and Raftery, A.E., Model-based Gaussian and non-Gaussian clustering. Biometrics. 1993; pp.803-821.
Cover, T.M. and Thomas, J.A., Information theory and statistics. Elements of information theory.1991;1(1), pp.279-335.
Biernacki, C., Celeux, G. and Govaert, G., Assessing a mixture model for clustering with the integrated completed likelihood. IEEE transactions on pattern analysis and machine intelligence. 2000;22(7), pp.719-725.
Linzer DA, Lewis JB. poLCA: An R package for polytomous variable latent class analysis. Journal of statistical software. 2011;42(1):1-29.
Venables WN, Ripley BD. Modern applied statistics with S-PLUS. Springer Science & Business Media; 2013.
Sinha P, Delucchi KL, Thompson BT, McAuley DF, Matthay MA, Calfee CS; NHLBI ARDS Network. Latent class analysis of ARDS subphenotypes: a secondary analysis of the statins for acutely injured lungs from sepsis (SAILS) study. Intensive Care Med. 2018;44:1859–1869.
Sinha P, Churpek MM, Calfee CS. Machine learning classifier models can identify acute respiratory distress syndrome phenotypes using readily available clinical data. Am J Respir Crit Care Med. 2020;202: 996–1004.
Channappanavar R, Perlman S. Pathogenic human coronavirus infections: causes and consequences of cytokine storm and immunopathology. Semin Immunopathol. 2017;39(5):529–39.
Lippi G, Favaloro EJ. D-dimer is associated with severity of coronavirus disease 2019: a pooled analysis. Thromb Haemost. 2020;120(5):876–8.
Cheng B, Hu J, Zuo X, Chen J, Li X, Chen Y, et al. Predictors of progression from moderate to severe coronavirus disease 2019: a retrospective cohort. Clin Microbiol Infect. 2020.
Dreher M, Kersten A, Bickenbach J, Balfanz P, Hartmann B, Cornelissen C, et al. The characteristics of 50 hospitalized COVID-19 patients with and without ARDS. Dtsch Arztebl Int. 2020;117(16):271–8.
Gao Y, Li T, HanM, Li X,Wu D, Xu Y, et al. Diagnostic utility of clinical laboratory data determinations for patients with the severe COVID-19. J Med Virol. 2020;92(7):791–6.
ShangW, Dong J, Ren Y, Tian M, Li W, Hu J, et al. The value of clinical parameters in predicting the severity of COVID-19. J Med Virol. 2020.
Sun Y, Dong Y,Wang L, Xie H, Li B, Chang C, et al. Characteristics and prognostic factors of disease severity in patients with COVID-19: the Beijing experience. J Autoimmun. 2020:102473.
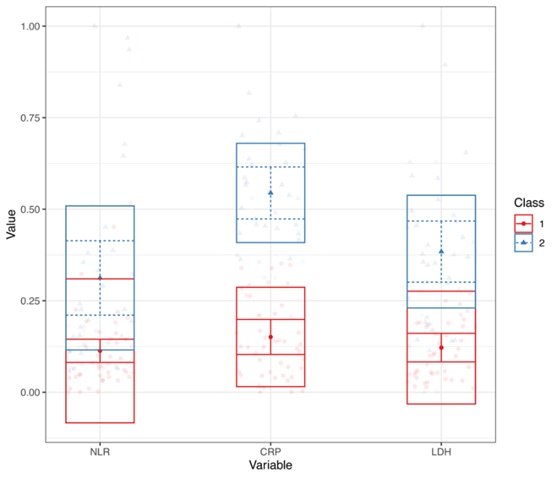
Downloads
Published
How to Cite
Issue
Section
License
Copyright (c) 2022 The Thai Society of Critical Care Medicine

This work is licensed under a Creative Commons Attribution-NonCommercial 4.0 International License.