Alzheimer’s Disease Classification and Prediction Using T1-weighted MR Brain Imaging Based on SVM Algorithm
Keywords:
Alzheimer’s Disease, Mild Cognitive Impairment, SVM Algorithm, T1-weighted MR imageAbstract
Introduction: Nowadays, Alzheimer’s disease (AD) is one of the worldwide health issues. Clinicians utilize Magnetic Resonance Brain imaging as one of the key biomarkers for AD diagnosis. Early-stage detection could prevent the high progression of the disease. The study aimed to create a machine learning model for predicting patients who are under an early stage of Alzheimer’s disease (AD) which were consisting of late mild cognitive impairment (LMCI), early mild cognitive impairment (EMCI), and cognitive normal (CN) for patient aged 65-75 using 3DT1-weighted MR Brain imaging based on Support Vector Machine (SVM) classification. Methods: The imaging data were acquired from the Alzheimer’s Disease Neuroimaging Initiative consisted of 61 LMCI patients, 95 EMCI patients, 92 cognitive normal subjects. There were three main steps of this work including 1) data preprocessing, 2) features extraction, and 3) algorithm classification. The first two steps were performed using FreeSurfer software to normalize the imaging data and extract features of interest. The final step was algorithm classification and algorithm training with three binary classification groups (CN vs. LMCI, CN vs. EMCI, and EMCI vs. LMCI) with feature selection training methodology based on F1-score, three classification models in total. Results: The CN vs. LMCI classifier achieved a 0.79 AUC value with 73.86% of accuracy. Meanwhile, the CN vs. EMCI classifier achieved a 0.64 AUC value with 59.89% of accuracy, and the EMCI vs. LMCI classifier achieved a 0.67 AUC value with 66.67% of accuracy. Conclusion: Our findings indicated that the proposed SVM classification models succeeded to classify and predict Alzheimer’s disease progression for CN vs. LMCI, EMCI vs. LMCI, and CN vs. EMCI ordered by its prediction performance from high to low, respectively. Importantly, we emphasized that MR Brain imaging might be a potential biomarker for an early-stage Alzheimer’s disease diagnosis.
References
2021 Alzheimer's disease facts and figures. Alzheimer's & Dementia. 2021;17(3):327-406.
Association As. 2019 Alzheimer's disease facts and figures. Alzheimer's & Dementia. 2019;15(3):321-87.
Sheng M, Sabatini BL, Südhof TC. Synapses and Alzheimer's disease. Cold Spring Harb Perspect Biol. 2012;4(5):a005777.
Bloom GS. Amyloid-β and Tau. JAMA Neurology. 2014;71(4):505.
Beason-Held LL, Goh JO, An Y, Kraut MA, O'Brien RJ, Ferrucci L, et al. Changes in Brain Function Occur Years before the Onset of Cognitive Impairment. Journal of Neuroscience. 2013;33(46):18008-14.
Jagust W. Imaging the evolution and pathophysiology of Alzheimer disease. Nature Reviews Neuroscience. 2018;19(11):687-700.
Doi K. Computer-aided diagnosis in medical imaging: Historical review, current status and future potential. Computerized Medical Imaging and Graphics. 2007;31(4-5):198-211.
Camlica Z, Tizhoosh HR, Khalvati F, editors. Medical Image Classification via SVM Using LBP Features from Saliency-Based Folded Data2015: IEEE.
Basaia S, Agosta F, Wagner L, Canu E, Magnani G, Santangelo R, et al. Automated classification of Alzheimer's disease and mild cognitive impairment using a single MRI and deep neural networks. NeuroImage: Clinical. 2019;21:101645.
Ding Y, Sohn JH, Kawczynski MG, Trivedi H, Harnish R, Jenkins NW, et al. A Deep Learning Model to Predict a Diagnosis of Alzheimer Disease by Using 18F-FDG PET of the Brain. Radiology. 2019;290(2):456-64.
Jongkreangkrai C, Vichianin Y, Tocharoenchai C, Arimura H. Computer-aided classification of Alzheimer's disease based on support vector machine with combination of cerebral image features in MRI. Journal of Physics: Conference Series. 2016;694:012036.
Tantiwetchayanon K, Vichianin Y, Ekjeen T, Srungboonmee K, Ngamsombat C, Chawalparit O. Comparison of the WEKA and SVM-light based on support vector machine in classifying Alzheimer’s disease using structural features from brain MR imaging. Journal of Physics: Conference Series. 2019;1248:012003.
(ADNI) AsDNI. Study design [Available from: http://adni.loni.usc.edu/study-design/#background-container.
Petersen RC, Aisen PS, Beckett LA, Donohue MC, Gamst AC, Harvey DJ, et al. Alzheimer's Disease Neuroimaging Initiative (ADNI): Clinical characterization. Neurology. 2010;74(3):201-9.
Fischl B. FreeSurfer. Neuroimage. 2012;62(2):774-81.
Rocha A, Goldenstein SK. Multiclass From Binary: Expanding One-Versus-All, One-Versus-One and ECOC-Based Approaches. IEEE Transactions on Neural Networks and Learning Systems. 2014;25(2):289-302.
Rifkin R, Klautau A. In Defense of One-Vs-All Classification. J Mach Learn Res. 2004;5:101–41.
Sasaki Y. The truth of the F-measure. Teach Tutor Mater. 2007.
Wood T. What is the F-score? [Available from: https://deepai.org/machine-learning-glossary-and-terms/f-score.
Hajian-Tilaki K. Receiver Operating Characteristic (ROC) Curve Analysis for Medical Diagnostic Test Evaluation. Caspian J Intern Med. 2013;4(2):627-35.
Chicco D, Jurman G. The advantages of the Matthews correlation coefficient (MCC) over F1 score and accuracy in binary classification evaluation. BMC Genomics. 2020;21(1).
Evans A, Collins L, Mills SR, Brown ED, Kelly RL, Peters T. 3D Statistical Neuroanatomical Models from 305 MRI Volumes1993. 1813-7 vol.3 p.
Mandal PK, Mahajan R, Dinov ID. Structural Brain Atlases: Design, Rationale, and Applications in Normal and Pathological Cohorts. Journal of Alzheimer's Disease. 2012;31(s3):S169-S88.
Figueroa RL, Zeng-Treitler Q, Kandula S, Ngo LH. Predicting sample size required for classification performance. BMC Medical Informatics and Decision Making. 2012;12(1):8.
Chen X, Harrison R, Zhang Y-Q. Multi-SVM Fuzzy Classification and Fusion Method and Applications in Bioinformatics. Journal of Computational and Theoretical Nanoscience. 2005;2:534-42.
Guernine T, Zeroual K, editors. SVM Fuzzy Hierarchical Classification Method for Multi-class Problems. 2009 International Conference on Advanced Information Networking and Applications Workshops; 2009 26-29 May 2009.
Hopper MW, Vogel FS. The limbic system in Alzheimer's disease. A neuropathologic investigation. Am J Pathol. 1976;85(1):1-20.
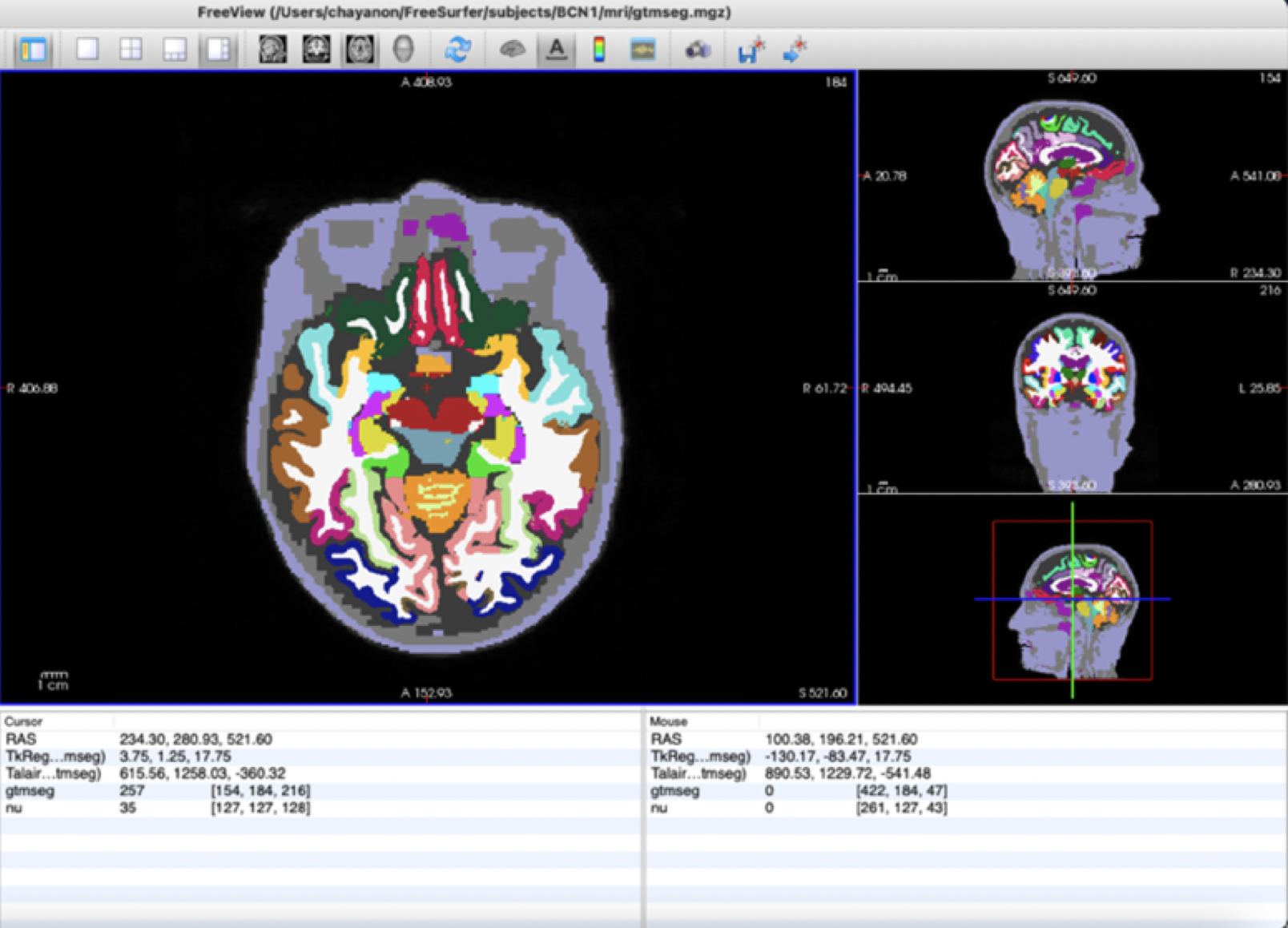
Downloads
Published
How to Cite
Issue
Section
License
บทความที่ได้รับการตีพิมพ์เป็นลิขสิทธิ์ของสมาคมรังสีเทคนิคแห่งประเทศไทย (The Thai Society of Radiological Technologists)
ข้อความที่ปรากฏในบทความแต่ละเรื่องในวารสารวิชาการเล่มนี้เป็นความคิดเห็นส่วนตัวของผู้เขียนแต่ละท่านไม่เกี่ยวข้องกับสมาคมรังสีเทคนิคแห่งประเทศไทยและบุคคลากรท่านอื่น ๆในสมาคม ฯ แต่อย่างใด ความรับผิดชอบองค์ประกอบทั้งหมดของบทความแต่ละเรื่องเป็นของผู้เขียนแต่ละท่าน หากมีความผิดพลาดใดๆ ผู้เขียนแต่ละท่านจะรับผิดชอบบทความของตนเองแต่ผู้เดียว