Local diagnostic reference levels for breast screening using digital mammography at Tanyawej Breast Center, Songklanagarind Hospital
Keywords:
Diagnostic reference levels, Average glandular dose, Entrance surface dose, Compress breast thicknessAbstract
Objective: To determine the local diagnostic reference levels (local DRL) for screening mammography at Tanyawej Breast Center, Songklanagarind Hospital on digital mammography Hologic Selenia dimensions.
Materials and Methods: Retrospective data of screening mammography were collected from 400 patients, 200 patients for 2D technique and 200 patients for Combo technique. The women ages between 40-75 years old who have the compressed breast thickness between 40-69 mm. between 1 January 2018 - 30 September 2019. The patient data and exposure parameters were collected as follows: average glandular dose (AGD, mGy); entrance surface air kerma (ESAK; mGy); compressed breast thickness (CBT, mm); compression force (CF, N); peak kilovoltage (kVp); tube current-time (mAs); target and filter (W/Rh, W/Ag); patient age (year); and patient positioning in terms of RCC, LCC, RMLO, LMLO were also recorded.
Results: The results showed that the AGD using compressed breast thickness in between 40-69 mm at the percentile 75 were 2.07 mGy and mGy for 2D technique and Combo-technique, respectively. The average glandular dose over the percentile 75 was 33% in 2D technique and Combo-technique.
Conclusion: The local DRLs for screening mammography using 2D and combo techniques obtained in this study were 2.07 mGy and 2.14 mGy, respectively.
Downloads
References
Joachim D, Sabine B, Johann Friedrich D. Segmentation of microcalcification in mammogram. IEEE Trans: Medical Imaging, 1993;(6): 34-642.
Richardson W.B., Apply wavelets to mammograms. IEEE Engineering: Medicine and Biology Magazine, 1995;(10):551-560.
Yala A, Schuster T, Barzilay R. A Deep Learning Mammography-based Model for Improved Breast Cancer Risk Prediction. Radiology, 2019;(292):60-66.
Valvano G, Santini G, Martini N, Ripoli A. Convolutional Neural Networks for the Segmentation of Microcalcification in Mammography Imaging. Journal of Healthcare Engineering, 2019.
Yala A, Schuster T, Barzilay R. A Deep Learning Mammography-based Model for Improved Breast Cancer Risk Prediction. Radiology, 2019;(292):60-66.
International Atomic Energy Agency, human health series no 17. Quality Assurance Program for digital mammography, 2011.
American College of Radiology. Mammography Quality Control Manual Mammography Phantom Image Quality Evaluation, 1999.
Mckinney SM, Sieniek M, Godbole V, Godwin J, Antropova N, Ashrafian H. International evaluation of an AI system for breast cancer screening. Nature, 2020;(557):89-94.
Moayyad E. Suleiman, Mark F. McEntee, Lucy Cartwright, Jennifer Diffey, Patrick C Brennan. Diagnostic reference levels for digital mammography in New South Wales. Journal of Medical Imaging and Radiation Oncology. 61; 2017. p. 48-57.
พิมพ์อร เจริญพานิช, ลักษมณ พิมลอัศวคุณ. การหาปริมาณรังสีอ้างอิงในการถ่ายภาพรังสีเต้านมด้วยระบบดิจิทัลของโรงพยาบาลจุฬาภรณ์ สภากาชาดไทย โดยศึกษาในกลุ่มประชากรที่แตกต่างกัน [วิทยานิพนธ์]. กรุงเทพฯ: จุฬาลงกรณ์มหาวิทยาลัย; 2562.
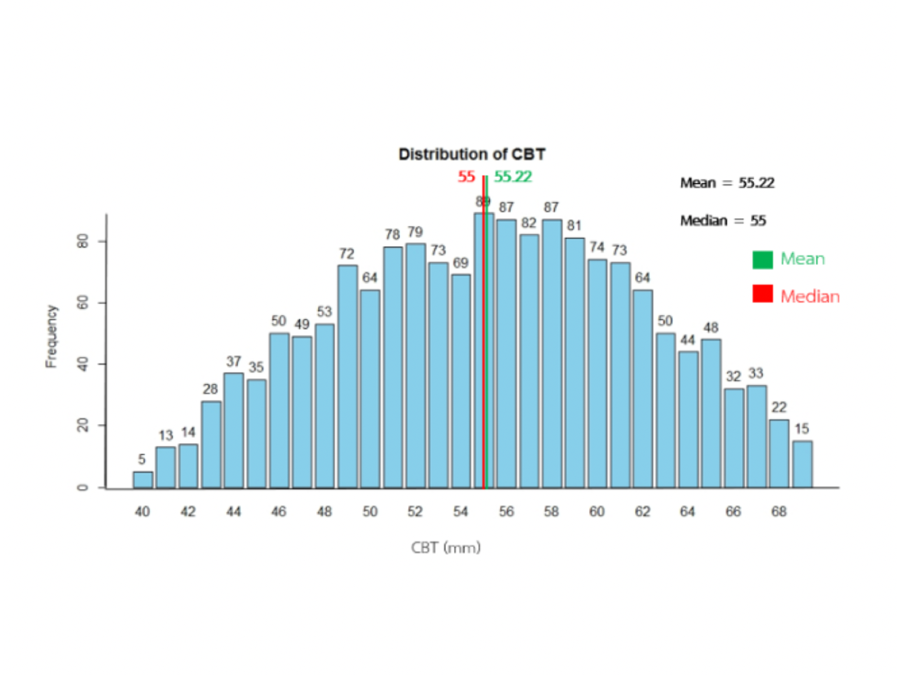
Downloads
Published
How to Cite
Issue
Section
License
บทความที่ได้รับการตีพิมพ์เป็นลิขสิทธิ์ของสมาคมรังสีเทคนิคแห่งประเทศไทย (The Thai Society of Radiological Technologists)
ข้อความที่ปรากฏในบทความแต่ละเรื่องในวารสารวิชาการเล่มนี้เป็นความคิดเห็นส่วนตัวของผู้เขียนแต่ละท่านไม่เกี่ยวข้องกับสมาคมรังสีเทคนิคแห่งประเทศไทยและบุคคลากรท่านอื่น ๆในสมาคม ฯ แต่อย่างใด ความรับผิดชอบองค์ประกอบทั้งหมดของบทความแต่ละเรื่องเป็นของผู้เขียนแต่ละท่าน หากมีความผิดพลาดใดๆ ผู้เขียนแต่ละท่านจะรับผิดชอบบทความของตนเองแต่ผู้เดียว