Diagnostic agreement between a commercial AI system and radiologists in interpreting checkup chest radiographs
Keywords:
artificial intelligence (AI) system, checkup chest radiograph (CXR), diagnostic agreementAbstract
Background: Chest radiography is widely used to screen for thoracic diseases. Recent artificial intelligence (AI) systems have demonstrated outstanding standalone performance in diagnostic tasks concerning chest radiographs (CXRs), often comparable to that of radiologists. However, few studies have evaluated AI performance in real-world clinical practice settings, especially with regard to checkup examinations and diagnostic agreement with humans. Objective: To evaluate the diagnostic agreement between AI and radiologists in evaluating checkup CXRs. Methods: An AI system and radiologists independently evaluated 500 checkup CXRs from a retrospective review period. We then quantified their diagnostic agreement. Results: When our analysis was restricted to lesions identified by the AI (AI-target lesions), we found fair agreement. If we regard the diagnoses of the radiologists as ground truth, the AI produced false-negative and false-positive rates of 8.0% and 8.7%, respectively. When extended to include lesions that had not been identified by the AI (both AI-target and AI-non-target lesions), our analysis showed a reduced agreement (76.0%) and an increased false-negative rate (16.7%). The AI demonstrated low sensitivity (26.5–36.8) but high specificity (90.1–90.5), with a significant number of AI-non-target lesions. Conclusions: Our results demonstrate fair to slight agreement between automatic AI diagnoses and the assessments of radiologists for checkup CXRs. Radiologists often reported numerous AI-non-target lesions. Despite certain limitations, our findings suggest that AI may play a valuable role as a diagnostic aid to enhance radiological evaluations. Further research is warranted to comprehensively assess AI performance in broader clinical contexts.
References
Lee JH, Sun HY, Park S, et al. Performance of a Deep Learning Algorithm Compared with Radiologic Interpretation for Lung Cancer Detection on Chest Radiographs in a Health Screening Population. Radiology. 2020;297(3):687-696. doi:10.1148/radiol. 2020201240
Yoo H, Kim EY, Kim H, et al. Artificial Intelligence-Based Identification of Normal Chest Radiographs: A Simulation Study in a Multicenter Health Screening Cohort. Korean J Radiol. 2022;23(10):1009-1018. doi:10.3348/kjr.2022.0189
Gampala S, Vankeshwaram V, Gadula SSP. Is Artificial Intelligence the New Friend for Radiologists? A Review Article. Cureus. 2020;12(10):e11137. Accessed October 24, 2020. doi:10.7759/cureus.11137
Siemens Healthcare GmbH. Operating Characteristics and Algorithm Performance AI-Rad Companion Chest X-ray Version 2.0 – 4th February 2021. Accessed January 1, 2022.
Ahn JS, Ebrahimian S, McDermott S, et al. Association of Artificial Intelligence-Aided Chest Radiograph Interpretation with Reader Performance and Efficiency. JAMA Netw Open. 2022;5(8):e2229289. Accessed August 1, 2022. doi:10.1001/jamane tworkopen.2022.29289
McHugh ML. Interrater reliability: the kappa statistic. Biochem Med (Zagreb). 2012;22(3):276-282.
Kim EY, Kim YJ, Choi WJ, et al. Performance of a deep-learning algorithm for referable thoracic abnormalities on chest radiographs: A multicenter study of a health screening cohort. PLoS One. 2021;16(2):e0246472. Accessed February 19, 2021. doi:10.1371/journal.pone.0246472
Khosangruang O, Chitranonth C. Radiographic findings of screening chest films in Priest Hospital. J Med Assoc Thai. 2008;91 Suppl 1:S21-S23.
Thammarach P, Khaengthanyakan S, Vongsurakrai S, et al. AI Chest 4 All. Annu Int Conf IEEE Eng Med Biol Soc. 2020;2020:1229-1233.doi:10.1109/EMBC44109.2020.9175 862
Qin ZZ, Sander MS, Rai B, et al. Using artificial intelligence to read chest radio graphs for tuberculosis detection: A multi-site evaluation of the diagnostic accuracy of three deep learning systems. Sci Rep. 2019;9(1):15000. Accessed October 18, 2019. doi:10.1038/s41598-019-51503-3
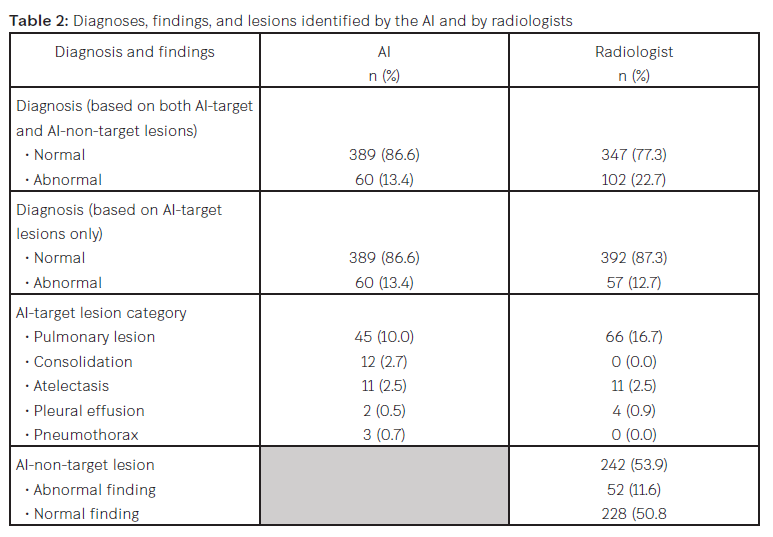
Downloads
Published
How to Cite
Issue
Section
License
Copyright (c) 2024 Chulabhorn Royal Academy

This work is licensed under a Creative Commons Attribution-NonCommercial-NoDerivatives 4.0 International License.
บทความที่ได้รับการตีพิมพ์เป็นลิขสิทธิ์ของราชวิทยาลัยจุฬาภรณ์
ข้อความที่ปรากฏในบทความแต่ละเรื่องในวารสารวิชาการเล่มนี้เป็นความคิดเห็นส่วนตัวของผู้เขียนแต่ละท่านไม่เกี่ยวข้องกับราชวิทยาลัยจุฬาภรณ์ และคณาจารย์ท่านอื่น ในราชวิทยาลัยฯ แต่อย่างใด ความรับผิดชอบองค์ประกอบทั้งหมดของบทความแต่ละเรื่องเป็นของผู้เขียนแต่ละท่าน หากมีความผิดพลาดใด ๆ ผู้เขียนแต่ละท่านจะรับผิดชอบบทความของตนเองแต่ผู้เดียว