The Intention to Use Telemedicine by Surgical Patients in Response to COVID-19
DOI:
https://doi.org/10.33192/Smj.2022.95Keywords:
Telemedicine, Covid 19, surgery, intention to useAbstract
Objective: This study explored patients’ intention to use telemedicine instead of traveling to a hospital during the current global COVID-19 crisis. The framework focused on the relationships between variables derived from the technology acceptance model and the extended unified theory of acceptance and use of technology model.
Materials and Methods: Multistage sampling procedures were applied to recruit samples using nonprobability sampling methods. Adult patients who had undergone surgery at a university hospital participated; all were experienced in using online meeting applications and online payment services in their daily lives. Consent forms and online questionnaires were distributed via a Google Forms link.
Results: Between October and December 2021, 502 patients undergoing procedures participated in the study. Five variables—social influence, trust, price, perceived usefulness, and perceived ease of use—significantly impacted intention to use. Perceived ease of use significantly impacted perceived usefulness, with a value of 0.679***. In addition, perceived ease of use indirectly influenced intention to use (impact value, 0.103***). Performance expectancy did not significantly impact intention to use, with an impact value of -0.012.
Conclusion: The contributions of this study will enable developers, medical professionals, and marketers to improve telemedicine services to better satisfy patients undergoing surgery and increase their intention to use telemedicine. However, the performance expectancy aspect may not warrant patients’ intention. Additionally, the research is recommended on other potential variables influencing telemedicine utilization, such as psychological expectations, performance expectations, and technical conditions.
References
Strehle EM, Shabde N. One hundred years of telemedicine: does this new technology have a place in paediatrics? Arch Dis Child. 2006;91:956-9.
Bullard JE. Contemporary glacigenic inputs to the dust cycle. 2013;38:71-89.
Costa D, Soares J, Lindert J, Hatzidimitriadou E, Sundin Ö, Toth O, et al. Intimate partner violence: a study in men and women from six European countries. Int J Public Health. 2015;60:467-78.
Urquhart AC, Antoniotti NM, Berg RL. Telemedicine--an efficient and cost-effective approach in parathyroid surgery. Laryngoscope. 2011;121:1422-5.
National Center for Immunization and Respiratory Diseases (NCIRD). 2020. [cited 27 January 2021]. Available from: https://www.cdc.gov/ncird/index.html
Chiiwii. chiiwiidoctor. 2021 [cited 27 January 2021]. Available from: https://www.chiiwiidoctor.com/
Seedoctornow. Seedoctornow. 2017. [cited 27 January 2021]. Available from: https://www.seedoctornow.com/
Ooca. Ooca It’s Okay. 2021. [cited 27 January 2021]. Available from: https://www.ooca.co/
Value Driven Care Unit. The number of actual used of siriraj telemedicine platform by patients with medical diseases Bangkok: Faculty of Medicine Siriraj Hospital, Value Driven Care Unit; 27 January 2021.
Razmak J, Bélanger C. Interdisciplinary Approach: A Lever to Business Innovation. Int J High Educ. 2016;5.
Bundot GY, Md Yunos J, Mohammed M. Technology Acceptance Model Of Intention To Use ICT By Academics In Nigerian Higher Education. Online Journal for TVET Practitioners. 2017;2:3-7.
Venkatesh V, Thong J, Xu X. Consumer Acceptance and Use of Information Technology: Extending the Unified Theory of Acceptance and Use of Technology. MIS Quarterly. 2012;36:157-78.
Naranjo-Zolotov M, Oliveira T, Casteleyn S. Citizens’ intention to use and recommend e-participation: Drawing upon UTAUT and citizen empowerment. Inf Technol People. 2018;32.
Kim HY. Statistical notes for clinical researchers: assessing normal distribution (2) using skewness and kurtosis. Restor Dent Endod. 2013;38:52-4.
George D, Mallery P. IBM SPSS Statistics 26 Step by Step: A Simple Guide and Reference 16th ed. New York: Routledge;2019.
Hair J, Hult GTM, Ringle C, Sarstedt M. A Primer on Partial Least Squares Structural Equation Modeling (PLS-SEM) 2022.
Campbell DT, Fiske DW. Convergent and discriminant validation by the multitrait-multimethod matrix. Psychol Bull. 1959;56:81-105.
Schmitt N, Stults DM. Methodology Review: Analysis of Multitrait-Multimethod Matrices. Appl Psychol Meas. 1986;10:1-22.
Hair.Jr. JF, Black. WC, Babin. BJ, Anderson. RE, L. Tatham. R. Multivariant Data Analysis. New Jersey: Pearson International Edition; 2006.
Alalwan AA, Dwivedi YK, Rana NP. Factors influencing adoption of mobile banking by Jordanian bank customers: Extending UTAUT2 with trust. Int J Inf Manage. 2017;37:99-110.
Blaise JH, Park JE, Bellas NJ, Gitchell TM, Phan V. Caffeine consumption disrupts hippocampal long-term potentiation in freely behaving rats. Physiol Rep. 2018;6(5):e13632.
Tan E, Lau J. Behavioural intention to adopt mobile banking among the millennial generation. Young Consum. 2016;17:18-31.
Ogunsola K, Fontaine R, Jan M. Impact of surface acting and deep acting techniques on teachers’ organizational commitment. PSU Research Review. 2020;4:61-79.
Luo X, Li H, Zhang J, Shim JP. Examining multi-dimensional trust and multi-faceted risk in initial acceptance of emerging technologies: An empirical study of mobile banking services. Decis Support Syst. 2010;49:222-34.
Venkatesh V, Morris MG, Davis GB, Davis FD. User acceptance of information technology: Toward a unified view. MIS Quarterly. 2003;27:425-78.
Afshan S, Sharif A. Acceptance of mobile banking framework in Pakistan. Telemed Inf. 2016;33:370-87.
Bhatiasevi V. An Extended UTAUT Model to Explain the Adoption of Mobile Banking. Inf Dev. 2016;32:799-814.
Oliveira T, Faria M, Thomas MA, Popovič A. Extending the understanding of mobile banking adoption: When UTAUT meets TTF and ITM. Int J Inf Manage. 2014;34:689-703.
Slade EL, Dwivedi YK, Piercy NC, Williams MD. Modeling consumers’ adoption intentions of remote mobile payments in the United Kingdom: Extending UTAUT with innovativeness, risk, and trust. Psychol Mark. 2015;32:860-73.
Khatun F, Palas MJU, Ray P. Using the Unified Theory of Acceptance and Use of Technology model to analyze cloud‑based mHealth service for primary care. Digit Med. 2017;3:69-75.
Park H, Chon Y, Lee J, Choi Ie J, Yoon KH. Service design attributes affecting diabetic patient preferences of telemedicine in South Korea. Telemed J E Health. 2011;17:442-51.
Cocosila M, Archer N. Perceptions of chronically ill and healthy consumers about electronic personal health records: a comparative empirical investigation. BMJ Open. 2014;4:e005304.
Lee MKO, Turban E. A Trust Model for Consumer Internet Shopping. Int J Electron Commer. 2001;6:75-91.
Dodds WB. In search of value: how price and store name information influence buyers' product perceptions. J Consum Mark. 1991;8:15-24.
Gupta S. Impact of Sales Promotions on when, what, and how much to buy. J Mark Res. 1988;25:342-55.
Rayport J, Sviokla J. Managing the marketspace. Harv Bus Rev. 1994;72:141-50.
Chen Y-H, Barnes S. Initial trust and online buyer behavior. Ind Manag Data Syst. 2007;107:21-36.
Eriksson K, Kerem K, Nilsson D. Customer acceptance of Internet banking in Estonia. Int J Bank Mark. 2005;23:200-16.
Guriting P, Ndubisi N. Borneo online banking: Evaluating customer perceptions and behavioural intention. Manag Res News. 2006;29:6-15.
Jaruwachirathanakul B, Fink D. Internet banking adoption strategies for a developing country: The case of Thailand. Internet Res. 2005;15:295-311.
Venkatesh V, Davis F. A Theoretical Extension of the Technology Acceptance Model: Four Longitudinal Field Studies. Manag Sci. 2000;46:186-204.
Davis F, Bagozzi R, Warshaw P. User Acceptance of Computer Technology: A Comparison of Two Theoretical Models. Manag Sci. 1989;35:982-1003.
Abbad M. E-banking in Jordan. Behav Inf Technol. 2013;32:681-94.
Çelik H. What determines Turkish customers’ acceptance of Internet banking? Int J Bank Mark. 2008;26:353-70.
Kesharwani A, Tripathy T. Dimensionality of Perceived Risk and Its Impact on Internet Banking Adoption: An Empirical Investigation. Serv Mark Q. 2012;33:177-93.
Lee M-C. Factors influencing the adoption of internet banking: An integration of TAM and TPB with perceived risk and perceived benefit. Electron Commer Res Appl 2009;8:130-41.
Wei W, Kim G, Miao L, Behnke C, Almanza B. Consumer inferences of corporate social responsibility (CSR) claims on packaged foods. J Bus Res. 2018;83:186-201.
Yuen YY, Yeow PH, Lim N. Internet banking acceptance in the United States and Malaysia: a cross-cultural examination. Mark Intell Plan. 2015; 33:292-308.
Kalinić Z, Liébana-Cabanillas FJ, Muñoz-Leiva F, Marinković V. The moderating impact of gender on the acceptance of peerto-peer mobile payment systems. Int J Bank Mark. 2020;38:138-58.
M. AAL, Alamro AS. Factors affecting intention to use e banking in Jordan. Int J Bank Mark 2019;38:86-112.
Leon S. Service mobile apps: a millennial generation perspective. Ind manage data syst. 2018;118:1-20.
Ozturk AB. Customer acceptance of cashless payment systems in the hospitality industry. Int J Contemp Hosp. 2016;28:801-17.
Zhao H, Sophia A, Lini Z, Nannapat J. Understanding the impact of financial incentives on near field communication (NFC) mobile payment adoption: an experimental analysis. Int J Bank Mark. 2019;37:1296-312.
Awang Z. Structural Equation Modeling Using Amos Graphic: UiTM Press; 2012.
Al-Mamary YH, Shamsuddin A. Testing of the Technology Acceptance Model in Context of Yemen. Mediterr J Soc Sci. 2015;6:268-73.
Sica C, Ghisi M. The Italian versions of the Beck Anxiety Inventory and the Beck Depression Inventory-II: Psychometric properties and discriminant power. 2007.p.27-50.
Wu J-H, Wang Y-M. Measuring KMS success: A respecification of the DeLone and McLean’s model. Inf Manag. 2006;43:728-39.
Bentler PM. Comparative fit indexes in structural models. Psychol Bull. 1990;107:238-46.
Sharma GP, Verma RC, Pathare P. Mathematical modeling of infrared radiation thin layer drying of onion slices. J Food Eng. 2005;71:282-6.
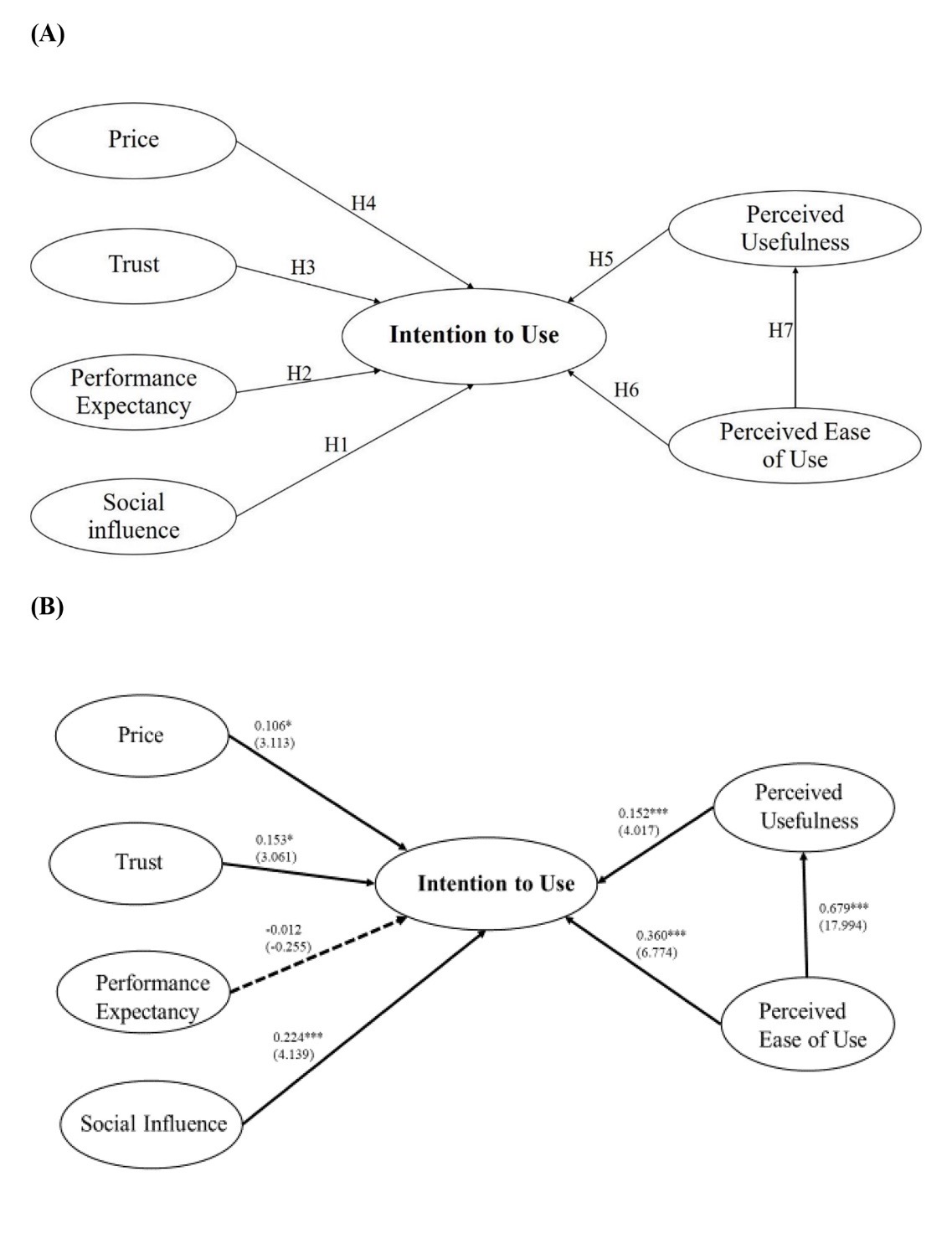
Published
How to Cite
Issue
Section
License

This work is licensed under a Creative Commons Attribution-NonCommercial-NoDerivatives 4.0 International License.
Authors who publish with this journal agree to the following conditions:
Copyright Transfer
In submitting a manuscript, the authors acknowledge that the work will become the copyrighted property of Siriraj Medical Journal upon publication.
License
Articles are licensed under a Creative Commons Attribution-NonCommercial-NoDerivatives 4.0 International License (CC BY-NC-ND 4.0). This license allows for the sharing of the work for non-commercial purposes with proper attribution to the authors and the journal. However, it does not permit modifications or the creation of derivative works.
Sharing and Access
Authors are encouraged to share their article on their personal or institutional websites and through other non-commercial platforms. Doing so can increase readership and citations.