Radiologist’s Role in Artificial Intelligence Era
DOI:
https://doi.org/10.33192/Smj.2022.104Keywords:
Artificial intelligence, performance, radiologist, radiologyAbstract
Artificial intelligence (AI) in radiology is recently a rapidly growing subject. Much literature about AI in radiology has been launched within 5 years, as well as commercial AI companies. This phenomenon makes some old radiologists feel worried about losing their jobs, and junior doctors hesitate to choose radiology as a specialty. Currently, implementations of proprietary AIs in clinical practice are limited, with a default setting for a convenient human overwrite. The AIs in clinical imaging largely remain either investigational as part of clinical/pre-clinical trials or being developed for commercialized purposes. Radiologists have an important role in all AI processes from the beginning to the end and vital in training the machine, as well as to validate its added benefit for outcome prediction/prognostication. This article will discuss the importance for radiologists to develop, implement, and monitor AI in clinical imaging, together with some ethical considerations. We would like to encourage radiologists to use AI as an adjunct tool, to save time and have better performance.
References
Gampala S, Vankeshwaram V, Gadula SSP. Is Artificial Intelligence the New Friend for Radiologists? A Review Article. Cureus. 2020;12(10):e11137.
Yakar D, Ongena YP, Kwee TC, Haan M. Do People Favor Artificial Intelligence Over Physicians? A Survey Among the General Population and Their View on Artificial Intelligence in Medicine. Value Health. 2022;25(3):374-81.
Soffer S, Ben-Cohen A, Shimon O, Amitai MM, Greenspan H, Klang E. Convolutional Neural Networks for Radiologic Images: A Radiologist’s Guide. Radiology. 2019;290(3):590-606.
Baltruschat I, Steinmeister L, Nickisch H, Saalbach A, Grass M, Adam G, et al. Smart chest X-ray worklist prioritization using artificial intelligence: a clinical workflow simulation. Eur Radiol. 2021;31(6):3837-45.
van Leeuwen KG, Schalekamp S, Rutten MJCM, van Ginneken B, de Rooij M. Artificial intelligence in radiology: 100 commercially available products and their scientific evidence. Eur Radiol. 2021;31(6):3797-804.
Pakdemirli E. Artificial intelligence in radiology: friend or foe? Where are we now and where are we heading? Acta Radiol Open. 2019;8(2):2058460119830222.
Rodriguez-Ruiz A, Lång K, Gubern-Merida A, Broeders M, Gennaro G, Clauser P, et al. Stand-Alone Artificial Intelligence for Breast Cancer Detection in Mammography: Comparison With 101 Radiologists. J Natl Cancer Inst. 2019;111(9):916-22.
Chartrand G, Cheng PM, Vorontsov E, Drozdzal M, Turcotte S, Pal CJ, et al. Deep Learning: A Primer for Radiologists. Radiographics. 2017;37(7):2113-31.
Sorantin E, Grasser MG, Hemmelmayr A, Tschauner S, Hrzic F, Weiss V, et al. The augmented radiologist: artificial intelligence in the practice of radiology. Pediatr Radiol [Internet]. 2021 Oct 19 [cited 2022 May 17]; Available from: https://doi.org/10.1007/s00247-021-05177-7
Jacques T, Fournier L, Zins M, Adamsbaum C, Chaumoitre K, Feydy A, et al. Proposals for the use of artificial intelligence in emergency radiology. Diagn Interv Imaging. 2021;102(2):63-8.
Wataganara T. Deep Learning for Nuchal Translucency–Based Screening for Fetal Trisomy 21. JAMA Netw Open. 2022;5(6):e2217869. doi:10.1001/jamanetworkopen.2022.17869
Cheng PM, Montagnon E, Yamashita R, Pan I, Cadrin-Chênevert A, Perdigón Romero F, et al. Deep Learning: An Update for Radiologists. RadioGraphics. 2021;41(5):1427-45.
Martín Noguerol T, Paulano-Godino F, Martín-Valdivia MT, Menias CO, Luna A. Strengths, Weaknesses, Opportunities, and Threats Analysis of Artificial Intelligence and Machine Learning Applications in Radiology. J Am Coll Radiol JACR. 2019;16(9 Pt B):1239-47.
van Leeuwen KG, de Rooij M, Schalekamp S, van Ginneken B, Rutten MJCM. How does artificial intelligence in radiology improve efficiency and health outcomes? Pediatr Radiol. 2022;52(11):2087-93.
Hosny A, Parmar C, Quackenbush J, Schwartz LH, Aerts HJWL. Artificial intelligence in radiology. Nat Rev Cancer. 2018;18(8):500-10.
Jones CM, Danaher L, Milne MR, Tang C, Seah J, Oakden-Rayner L, et al. Assessment of the effect of a comprehensive chest radiograph deep learning model on radiologist reports and patient outcomes: a real-world observational study. BMJ Open. 2021;11(12):e052902.
Strohm L, Hehakaya C, Ranschaert ER, Boon WPC, Moors EHM. Implementation of artificial intelligence (AI) applications in radiology: hindering and facilitating factors. Eur Radiol. 2020;30(10):5525-32.
Waymel Q, Badr S, Demondion X, Cotten A, Jacques T. Impact of the rise of artificial intelligence in radiology: What do radiologists think? Diagn Interv Imaging. 2019;100(6):327-36.
Rezazade Mehrizi MH, van Ooijen P, Homan M. Applications of artificial intelligence (AI) in diagnostic radiology: a technography study. Eur Radiol. 2021;31(4):1805-11.
Wataganara T, Rekhawasin T, Sompagdee N, Viboonchart S, Phithakwatchara N, Nawapun K. A 10-Year Retrospective Review of Prenatal Applications, Current Challenges and Future Prospects of Three-Dimensional Sonoangiography. Diagnostics (Basel). 2021;11(8):1511.
Pankhania M. Artificial intelligence and radiology: Combating the COVID-19 conundrum. Indian J Radiol Imaging. 2021;31(Suppl 1):S4-10.
Mallio CA, Quattrocchi CC, Beomonte Zobel B, Parizel PM. Artificial intelligence, chest radiographs, and radiology trainees: a powerful combination to enhance the future of radiologists? Quant Imaging Med Surg. 2021;11(5):2204-7.
Di Basilio F, Esposisto G, Monoscalco L, Giansanti D. The Artificial Intelligence in Digital Radiology: Part 2: Towards an Investigation of acceptance and consensus on the Insiders. Healthc Basel Switz. 2022;10(1):153.
Sand M, Durán JM, Jongsma KR. Responsibility beyond design: Physicians’ requirements for ethical medical AI. Bioethics. 2022;36(2):162-9.
Kim B, Koopmanschap I, Mehrizi MHR, Huysman M, Ranschaert E. How does the radiology community discuss the benefits and limitations of artificial intelligence for their work? A systematic discourse analysis. Eur J Radiol. 2021;136:109566.
Poursabzi-Sangdeh F, Goldstein DG, Hofman JM, Vaughan JW, Wallach H. Manipulating and Measuring Model Interpretability [Internet]. arXiv; 2021 Aug [cited 2022 May 17]. Report No.:arXiv:1802.07810. Available from: http://arxiv.org/abs/1802.07810
Mazurowski MA. Artificial Intelligence in Radiology: Some Ethical Considerations for Radiologists and Algorithm Developers. Acad Radiol. 2020;27(1):127-9.
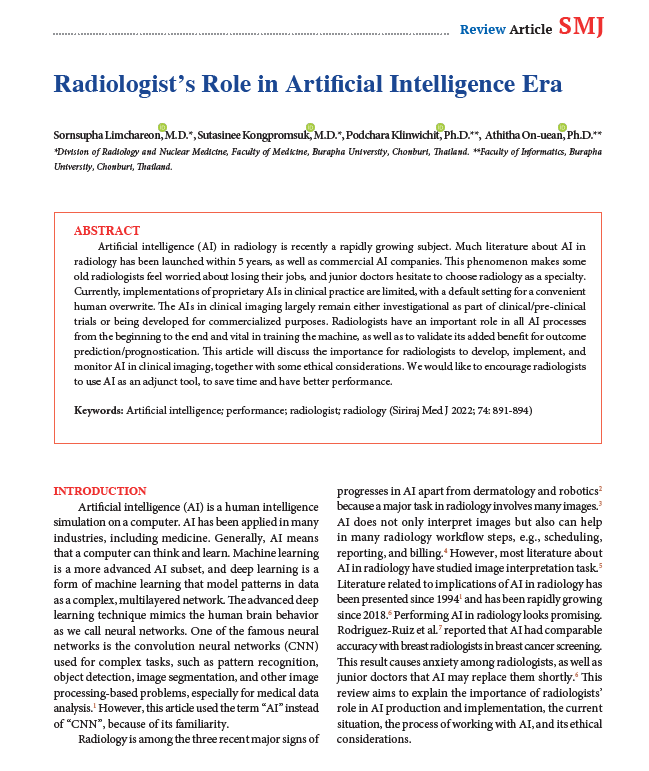
Published
How to Cite
Issue
Section
License

This work is licensed under a Creative Commons Attribution-NonCommercial-NoDerivatives 4.0 International License.
Authors who publish with this journal agree to the following conditions:
Copyright Transfer
In submitting a manuscript, the authors acknowledge that the work will become the copyrighted property of Siriraj Medical Journal upon publication.
License
Articles are licensed under a Creative Commons Attribution-NonCommercial-NoDerivatives 4.0 International License (CC BY-NC-ND 4.0). This license allows for the sharing of the work for non-commercial purposes with proper attribution to the authors and the journal. However, it does not permit modifications or the creation of derivative works.
Sharing and Access
Authors are encouraged to share their article on their personal or institutional websites and through other non-commercial platforms. Doing so can increase readership and citations.