Beyond Vision: Potential Role of AI-enabled Ocular Scans in the Prediction of Aging and Systemic Disorders
Role of AI-enabled Ocular Scans in the Prediction of Aging and Systemic Disorders
DOI:
https://doi.org/10.33192/smj.v76i2.266303Keywords:
fundus autofluorescence, ROPtool, Convolutional Neural Network (CNN), Color Fundus Photography (CFP), Machine Learning (ML), AI-Driven ocular scans, Area under the curve (AUC), deep learning, retinal age (RA)Abstract
In all medical subfields, including ophthalmology, the development of artificial intelligence (AI), particularly cutting-edge deep learning frameworks, has sparked a quiet revolution. The eyes and the rest of the body are anatomically related because of the unique microvascular and neuronal structures they possess. Therefore, ocular image-based AI technology may be a helpful substitute or extra screening method for systemic disorders, particularly in areas with limited resources. This paper provides an overview of existing AI applications for the prediction of systemic diseases from multimodal ocular pictures, including retinal diseases, neurological diseases, anemia, chronic kidney disease, autoimmune diseases, sleep disorders, cardiovascular diseases, and various others. It also covers the process of aging and its predictive biomarkers obtained from AI-based retinal scans. Finally, we also go through these applications existing problems and potential future paths.
References
He J, Baxter SL, Xu J, Xu J, Zhou X, Zhang K. The practical implementation of artificial intelligence technologies in medicine. Nat Med. 2019;25(1):30-6.
Zhang B, Chou Y, Zhao X, Yang J, Chen Y. Early detection of microvascular impairments with optical coherence tomography angiography in diabetic patients without clinical retinopathy: a meta-analysis. Am J Ophthalmol. 2021;222:226-37.
Moraes G, Fu DJ, Wilson M, Khalid H, Wagner SK, Korot E, et al. Quantitative analysis of OCT for neovascular age-related macular degeneration using deep learning. Ophthalmology. 2021;128(5):693-705.
Campbell JP, Kim SJ, Brown JM, Ostmo S, Chan RVP, Kalpathy-Cramer J, et al. Evaluation of a deep learning-derived quantitative retinopathy of prematurity severity scale. Ophthalmology. 2021;128(7):1070-6.
Xiangyu C, Yanwu X, Damon Wing Kee W, Tien Yin W, Jiang L. Glaucoma detection based on deep convolutional neural network. Annu Int Conf IEEE Eng Med Biol Soc. 2015;2015:715-8.
Hamet P, Tremblay J. Artificial intelligence in medicine. Metabolism 2017;69S:S36-S40.
Ramesh AN, Kambhampati C, Monson JR, Drew PJ. Artificial intelligence in medicine. Ann R Coll Surg Engl. 2004;86(5):334-8.
Chamie J. Population ageing: An inescapable future. Global Issues. [cited 2023 July 23]. Available from: https://www.globalissues.org/news/2022/01/05/29746
Childs BG, Durik M, Baker DJ, van Deursen JM. Cellular senescence in aging and age-related disease: from mechanisms to therapy. Nat Med. 2015;21(12):1424-35.
Lowsky DJ, Olshansky SJ, Bhattacharya J, Goldman DP. Heterogeneity in healthy aging. J Gerontol A Biol Sci Med Sci 2014;69(6):640-9.
Jylhävä J, Pedersen NL, Hägg S. Biological Age Predictors. EBioMedicine. 2017;21:29-36.
Horvath S, Raj K. DNA methylation-based biomarkers and the epigenetic clock theory of ageing. Nat Rev Genet. 2018;19:371-84.
Wang J, Knol MJ, Tiulpin A, Dubost F, de Bruijne M, Vernooij MW, et al. Gray matter age prediction as a biomarker for risk of dementia. Proc Natl Acad Sci U S A. 2019;116:21213-8.
Xia X, Chen X, Wu G, Li F, Wang Y, Chen Y, et al. Three-dimensional facial-image analysis to predict heterogeneity of the human ageing rate and the impact of lifestyle. Nat Metab 2020;2:946-57.
Cole JH, Ritchie SJ, Bastin ME, Valdes Hernandez MC, Munoz Maniega S, Royle N, et al. Brain age predicts mortality. Mol Psychiatry. 2018;23:1385-92.
Country MW. Retinal metabolism: A comparative look at energetics in the retina. Brain Res. 2017;1672:50-57.
Ikram MK, Ong YT, Cheung CY, Wong TY. Retinal vascular caliber measurements: clinical significance, current knowledge and future perspectives. Ophthalmologica. 2013;229(3):125-36.
Zhu Z, Shi D, Guankai P, Tan Z, Shang X, Hu W, et al. Retinal age gap as a predictive biomarker for mortality risk. Br J Ophthalmol. 2023;107:547-54.
Nusinovici S, Rim TH, Yu M, Lee G, Tham YC, Cheung N, et al. Retinal photograph-based deep learning predicts biological age, and stratifies morbidity and mortality risk. Age Ageing. 2022;51(4):afac065.
Ahadi S, Wilson KA, Babenko B, McLean CY, Bryant D, Pritchard O, et al. Longitudinal fundus imaging and its genome-wide association analysis provide evidence for a human retinal aging clock. Elife. 2023;12:e82364.
Arrigo A, Aragona E, Bandello F. Artificial intelligence for the diagnosis and screening of retinal diseases. touchReview in Ophthalmology. 2023;17(2):9-14. https://doi.org/10.17925/usor.2023.17.2.1
Hansen MB, Abràmoff MD, Folk JC, Mathenge W, Bastawrous A, Peto T. Results of automated retinal image analysis for detection of diabetic retinopathy from the Nakuru study, Kenya. PLoS One. 2015;10(10):e0139148.
Abràmoff MD, Folk JC, Han DP, Walker JD, Williams DF, Russell SR, et al. Automated analysis of retinal images for detection of referable diabetic retinopathy. JAMA Ophthalmol. 2013;131(3):351-7.
US Food & Drug Administration. FDA permits marketing of artificial intelligence-based device to detect certain diabetes-related eye problems. News release, April 2018. DOI: 10.31525/fda2-ucm604357.htm.
Ribeiro L, Oliveira CM, Neves C, Ramos JD, Ferreira H, Cunha-Vaz J. Screening for diabetic retinopathy in the central region of Portugal. Added value of automated ‘disease/no disease’ grading. Ophthalmologica. 2014 Nov 26.
Tufail A, Kapetanakis VV, Salas-Vega S, Egan C, Rudisill C, Owen CG, et al. An observational study to assess if automated diabetic retinopathy image assessment software can replace one or more steps of manual imaging grading and to determine their cost-effectiveness. Health Technol Assess. 2016;20(92):1-72.
Solanki K, Ramachandra C, Bhat S, Bhaskaranand M, Nittala MG, Sadda SR. Eyeart: Automated, high-throughput, image analysis for diabetic retinopathy screening. Investtigative Ophthalmology & Visual Science. 2015;56:1429.
Rajalakshmi R, Subashini R, Anjana RM, Mohan V. Automated diabetic retinopathy detection in smartphone-based fundus photography using artificial intelligence. Eye (Lond). 2018;32(6):1138-44.
Heydon P, Egan C, Bolter L, Chambers R, Anderson J, Aldington S, et al. Prospective evaluation of an artificial intelligence-enabled algorithm for automated diabetic retinopathy screening of 30,000 patients. Br J Ophthalmol. 2021;105(5):723-8.
Gulshan V, Peng L, Coram M, Stumpe MC, Wu D, Narayanaswamy A, et al. Development and validation of a deep learning algorithm for detection of diabetic retinopathy in retinal fundus photographs. JAMA. 2016;316(22):2402-10.
Ting DSW, Cheung CY-L, Lim G, Tan GSW, Quang ND, Gan A, et al. Development and validation of a deep learning system for diabetic retinopathy and related eye diseases using retinal images from multiethnic populations with diabetes. JAMA. 2017;318(22):2211-23.
Lin D, Xiong J, Liu C, Zhao L, Li Z, Yu S, et al. Application of comprehensive artificial intelligence retinal expert (CARE) system: A national real-world evidence study. Lancet Digit Health. 2021;3(8):e486-e95.
Lee CS, Baughman DM, Lee AY. Deep learning is effective for the classification of OCT images of normal versus age-related macular degeneration. Ophthalmol Retina. 2017;1(4):322-7.
Lee CS, Tyring AJ, Deruyter NP, Wu Y, Rokem A, Lee AY, et al. Deep-learning based, automated segmentation of macular edema in optical coherence tomography. Biomed Opt Express. 2017;8(7):3440-8.
Moraes G, Fu DJ, Wilson M, Khalid H, Wagner SK, Korot E, et al. Quantitative analysis of OCT for neovascular age-related macular degeneration using deep learning. Ophthalmology. 2021;128(5):693-705.
Waldstein SM, Vogl W-D, Bogunovic H, Sadeghipour A, Riedl S, Schmidt-Erfurth U. Characterization of drusen and hyperreflective foci as biomarkers for disease progression in age-related macular degeneration using artificial intelligence in optical coherence tomography. JAMA Ophthalmol. 2020;138(7):740-7.
Schmidt-Erfurth U, Reiter GS, Riedl S, Seebock P, Vogl WD, Blodi BA, et al. AI-based monitoring of retinal fluid in disease activity and under therapy. Prog Retin Eye Res. 2022;86:100972.
Bogunović H, Mares V, Reiter GS, Schmidt-Erfurth U. Predicting treat-and-extend outcomes and treatment intervals in neovascular age-related macular degeneration from retinal optical coherence tomography using artificial intelligence. Front Med (Lausanne). 2022;9:958469.
Holomcik D, Seeböck P, Gerendas BS, Mylonas G, Najeeb BH, Schmidt-Erfurth U, et al. Segmentation of macular neovascularization and leakage in fluorescein angiography images in neovascular age-related macular degeneration using deep learning. Eye (Lond). 2023;37(7):1439-44.
Vogl W-D, Riedl S, Mai J, Reiter GS, Lachinov D, Bogunovic H, et al. Predicting topographic disease progression and treatment response of pegcetacoplan in geographic atrophy quantified by deep learning. Ophthalmology Retina. 2023;7(1):4-13.
Liu Y, Holekamp NM, Heier JS. Prospective, Longitudinal study: Daily self-imaging with home OCT for neovascular age-related macular degeneration. Ophthalmol Retina. 2022;6(7):575-85.
Mathai M, Reddy S, Elman MJ, Garfinkel RA, Ladd B, Wagner AL, et al. Analysis of the long-term visual outcomes of ForeseeHome remote telemonitoring: The ALOFT study. Ophthalmol Retina. 2022;6(10):922-9.
Wang H, Abbas KM, Abbasifard M, Abbasi-Kangevari M, Abbastabar H, Abd-Allah F, et al. Global age-sex-specific fertility, mortality, healthy life expectancy (HALE), and population estimates in 204 countries and territories, 1950-2019: a comprehensive demographic analysis for the global burden of disease study 2019. Lancet. 2020;396(10258):1160-203.
Hannappe MA, Arnould L, Méloux A, Mouhat B, Bichat F, Zeller M, et al. Vascular density with optical coherence tomography angiography and systemic biomarkers in low and high cardiovascular risk patients. Sci Rep. 2020;10(1):16718.
Poplin R, Varadarajan AV, Blumer K, Liu Y, McConnell MV, Corrado GS, et al. Prediction of cardiovascular risk factors from retinal fundus photographs via deep learning. Nat Biomed Eng. 2018;2(3):158–64.
Kim YD, Noh KJ, Byun SJ, Lee S, Kim T, Sunwoo L, et al. Effects of hypertension, diabetes, and smoking on age and sex prediction from retinal fundus images. Sci Rep. 2020;10(1):4623.
Son J, Shin JY, Chun EJ, Jung KH, Park KH, Park SJ. Predicting high coronary artery calcium score from retinal fundus images with deep learning algorithms. Transl Vision Sci Technol. 2020;9(2):28.
Kim YD, Noh KJ, Byun SJ, Lee S, Kim T, Sunwoo L, et al. Effects of hypertension, diabetes, and smoking on age and sex prediction from retinal fundus images. Sci Rep. 2020;10(1):4623.
Son J, Shin JY, Chun EJ, Jung KH, Park KH, Park SJ. Predicting high coronary artery calcium score from retinal fundus images with deep learning algorithms. Transl Vision Sci Technol. 2020;9(2):28.
Chang J, Ko A, Park SM, Choi S, Kim K, Kim SM, et al. Association of cardiovascular mortality and deep learning-funduscopic atherosclerosis score derived from retinal fundus images. Am J Ophthalmol. 2020;217:121-30.
Zhang K, Liu X, Xu J, Yuan J, Cai W, Chen T, et al. Deep-learning models for the detection and incidence prediction of chronic kidney disease and type 2 diabetes from retinal fundus images. Nat Biomed Eng. 2021;5(6):533-45.
Babenko B, Traynis I, Chen C, Singh P, Uddin A, Cuadros J, et al. A deep learning model for novel systemic biomarkers in photographs of the External Eye: A Retrospective Study. Lancet Digit Health. 2023;5(5):e257-64.
Cheung CY, Ran AR, Wang S, Chan VTT, Sham K, Hilal S, et al. A deep learning model for detection of Alzheimer’s disease based on retinal photographs: a retrospective, multicentre case-control study. Lancet Digit Health. 2022;4(11):e806-15.
O’Bryhim BE, Lin JB, Stavern GPV, Apte RS. OCT angiography findings in preclinical Alzheimer’s disease: 3-year follow-up. Ophthalmology. 2021;128(10):1489-91.
Nunes A, Silva G, Duque C, Januario C, Santana I, Ambrosio AF, et al. Retinal texture biomarkers may help to discriminate between Alzheimer’s, Parkinson’s, and healthy controls. PLoS ONE. 2019;14(6):e0218826.
Wisely CE, Wang D, Henao R, Grewal DS, Thompson AC, Robbins CB, et al. Convolutional neural network to identify symptomatic Alzheimer’s disease using multimodal retinal imaging. Br J Ophthalmol. 2022;106(3):388-95.
Wong CW, Wong TY, Cheng CY, Sabanayagam C. Kidney and eye diseases: common risk factors, etiological mechanisms, and pathways. Kidney Int. 2014;85(6):1290-302.
Wong TY, Coresh J, Klein R, Muntner P, Couper DJ, Sharrett AR, et al. Retinal microvascular abnormalities and renal dysfunction: the atherosclerosis risk in communities study. J Am Soc Nephrol. 2004;15(9):2469-76.
Pakzad-Vaezi K, Pepple KL. Tubulointerstitial nephritis and uveitis. Curr Opin Ophthalmol. 2017;28(6):629-35.
Liew G, Mitchell P, Wong TY, Iyengar SK, Wang JJ. CKD increases the risk of age-related macular degeneration. J Am Soc Nephrol. 2008;19(4):806-11.
Kang EY, Hsieh YT, Li CH, Huang YJ, Kuo CF, Kang JH, et al. Deep learning-based detection of early renal function impairment using retinal fundus images: model development and validation. JMIR Med Inform. 2020;8(11):e23472.
Sabanayagam C, Xu D, Ting DSW, Nusinovici S, Banu R, Hamzah H, et al. A deep learning algorithm to detect chronic kidney disease from retinal photographs in community-based populations. Lancet Digit Health. 2020;2(6):e295-302.
Bauskar S, Jain P, Gyanchandani M. A Noninvasive Computerized Technique to Detect Anemia Using Images of Eye Conjunctiva. Pattern Recognit. Image Anal. 2019;29:438-46.
Chen Y, Zhong K, Zhu Y, Sun Q. Two-Stage Hemoglobin Prediction Based on Prior Causality. Front Public Health. 2022;10:1079389.
Mitani A, Huang A, Venugopalan S, Corrado GS, Peng L, Webster DR, et al. Detection of Anaemia from Retinal Fundus Images via Deep Learning. Nat Biomed Eng. 2020;4(1):18-27.
Zhao X, Meng L, Su H, Lv B, Lv C, Xie G, et al. Deep-Learning-Based Hemoglobin Concentration Prediction and Anemia Screening Using Ultra-Wide Field Fundus Images. Front Cell Dev Biol. 2022;10:888268.
Wei H, Shen H, Li J, Zhao R, Chen Z. AneNet: A Lightweight Network for the Real-Time Anemia Screening from Retinal Vessel Optical Coherence Tomography Images. Optics & Laser Technology. 2020;136(1):106773.
Wu Y, Wang D, Wu X, Shen L, Zhao L, Wang W, et al. Optical Coherence Tomography Angiography for the Characterisation of Retinal Microvasculature Alterations in Pregnant Patients with Anaemia: A Nested Case-control Study. Br J Ophthalmol. 2022:bjophthalmol-2022-321781.
Graves J, Balcer LJ. Eye disorders in patients with multiple sclerosis: natural history and management. Clin Ophthalmol. 2010;4:1409-22.
Garcia-Martin E, Ortiz M, Boquete L, Sánchez-Morla EM, Barea R, Cavaliere C, et al. Early diagnosis of multiple sclerosis by OCT analysis using Cohen’s d method and a neural network as classifier. Comput Biol Med. 2021;129:104165.
Peng CY, Chien RN, Liaw YF. Hepatitis B virus-related decompensated liver cirrhosis: benefits of antiviral therapy. J Hepatol. 2012;57(2):442-50.
Xiao W, Huang X, Wang JH, Lin DR, Zhu Y, Chen C, et al. Screening and identifying hepatobiliary diseases through deep learning using ocular images: A prospective, multicentre study. Lancet Digit Health. 2021;3(2):e88-e97.
Reichenbach A, Fuchs U, Kasper M, el-Hifnawi E, Eckstein AK. Hepatic retinopathy: morphological features of retinal glial (Müller) cells accompanying hepatic failure. Acta Neuropathol. 1995;90(3):273-81.
Onder C, Bengur T, Selcuk D, Bulent S, Belkis U, Ahmet M, et al. Relationship between retinopathy and cirrhosis. World J Gastroenterol. 2005;11(14):2193-6.
Iwakiri Y. Pathophysiology of portal hypertension. Clin Liver Dis. 2014;18(2):281-91.
Asrani SK, Devarbhavi H, Eaton J, Kamath PS. Burden of liver diseases in the world. J Hepatol. 2019;70(1):151-71.
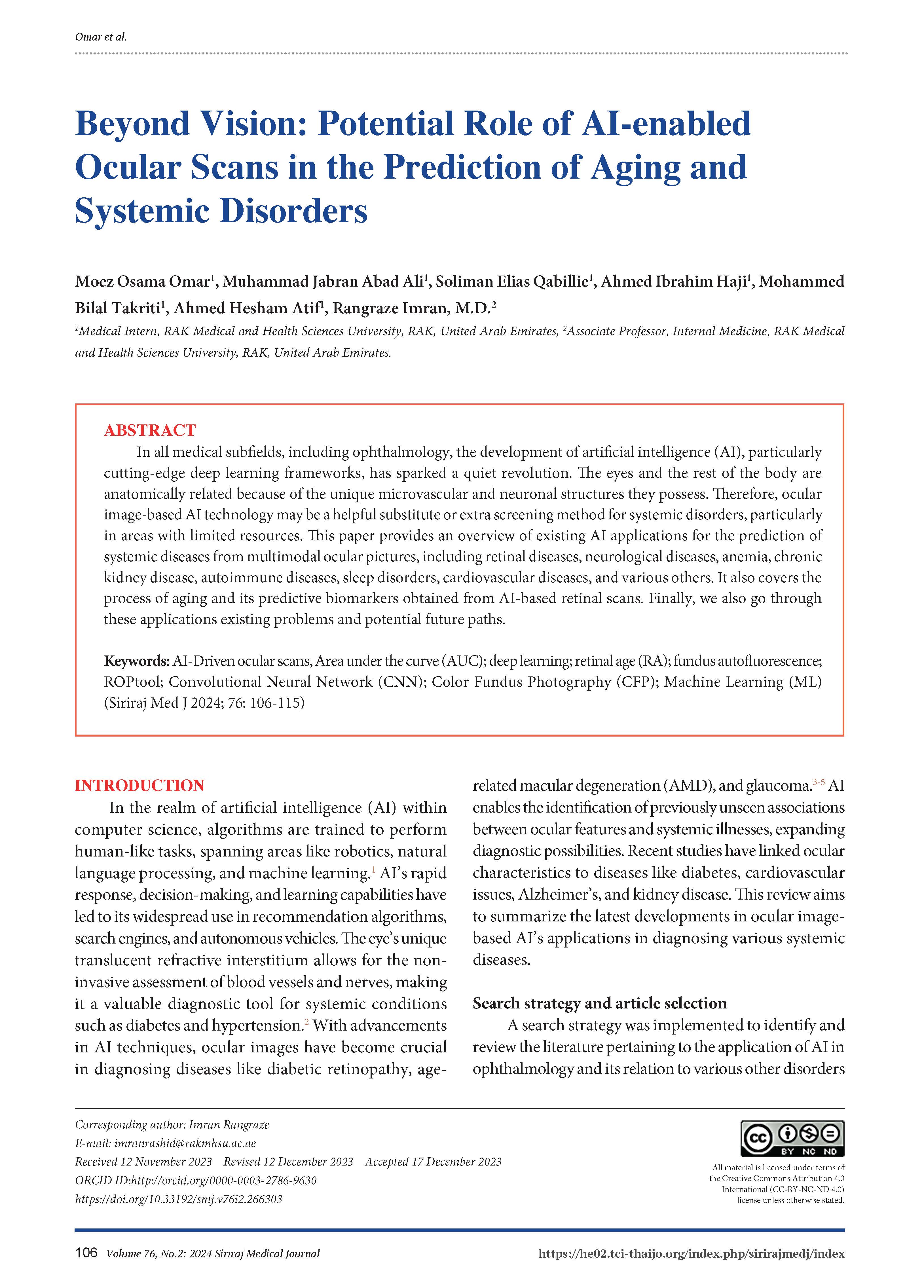
Published
How to Cite
License
Copyright (c) 2024 Siriraj Medical Journal

This work is licensed under a Creative Commons Attribution-NonCommercial-NoDerivatives 4.0 International License.
Authors who publish with this journal agree to the following conditions:
Copyright Transfer
In submitting a manuscript, the authors acknowledge that the work will become the copyrighted property of Siriraj Medical Journal upon publication.
License
Articles are licensed under a Creative Commons Attribution-NonCommercial-NoDerivatives 4.0 International License (CC BY-NC-ND 4.0). This license allows for the sharing of the work for non-commercial purposes with proper attribution to the authors and the journal. However, it does not permit modifications or the creation of derivative works.
Sharing and Access
Authors are encouraged to share their article on their personal or institutional websites and through other non-commercial platforms. Doing so can increase readership and citations.