Development of Machine Learning Algorithms for Predicting Preoperative and Postoperative venous Thromboembolism in Patients Undergoing Surgery for Spinal Metastasis
DOI:
https://doi.org/10.33192/smj.v76i6.266959Keywords:
Development, machine-learning algorithms, prediction, venous thromboembolism, surgery, spinal metastasisAbstract
Objective: This study aims to develop and compare machine learning models (MLMs) for predicting venous thromboembolism (VTE) in patients undergoing surgery for spinal metastasis. The study evaluates the predictive capabilities of MLMs for preoperative and postoperative VTE within different time frames.
Materials and Methods: A total of 334 patients undergoing surgery for spinal metastasis were included, with a mean age of 57.6 years and 57.2% being male. The investigation assessed postoperative VTE prevalence within 30 and 90 days, with pulmonary embolism (PE) and deep vein thrombosis (DVT) rates at 20% and 80%, respectively. Key patient-related factors—age, body mass index, preoperative ambulatory status, albumin level, hemoglobin level, partial thromboplastin time, and operative time—were considered potential predictors of VTE.
Results: The postoperative VTE prevalence was 8.98% within 30 days and 13.47% within 90 days. Age, body mass index, preoperative ambulatory status, albumin level, hemoglobin level, partial thromboplastin time, and operative time emerged as significant VTE predictors. The gradient boosted tree algorithm was the best-performing MLM for predicting VTE within 90 days, with AUC values of 0.77 preoperatively and 0.71 postoperatively. For predicting VTE within 30 days, the support vector machine model was most effective, with AUCs of 0.72 preoperatively and 0.68 postoperatively.
Conclusion: Predictive analytics and MLMs effectively predict preoperative and postoperative VTE in patients undergoing surgery for spinal metastasis. Identified key factors and MLM performance metrics offer valuable insights for risk assessment and preventive measures in this patient population.
References
Walker AJ, Card TR, West J, Crooks C, Grainge MJ. Incidence of venous thromboembolism in patients with cancer–a cohort study using linked United Kingdom databases. Eur J Cancer. 2013;49(6):1404-13.
Heit JA, Silverstein MD, Mohr DN Petterson TM, O'Fallon WM, Melton LJ 3rd. Risk factors for deep vein thrombosis and pulmonary embolism: a population-based case-control study. Arch Intern Med. 2000;160(6):809-15.
Gussoni G, Frasson S, La Regina M, Di Micco P, Monreal M, RIETE Investigators. Three-month mortality rate and clinical predictors in patients with venous thromboembolism and cancer.Findings from the RIETE registry.Thromb Res. 2013;131(1):24-30.
Zhang L, Cao H, Chen Y, Jiao G. Risk factors for venous thromboembolism following spinal surgery: A meta-analysis. Medicine (Baltimore). 2020;99(29):e20954.
Zacharia BE, Kahn S, Bander ED, Cederquist GY, Cope WP, McLaughlin L, et al. Incidence and risk factors for preoperative deep venous thrombosis in 314 consecutive patients undergoing surgery for spinal metastasis. J Neurosurg Spine. 2017;27(2):189-97.
Groot OQ, Ogink PT, Pereira NRP, Ferrone ML, Harris MB, Lozano-Calderon SA, et al. High risk of symptomatic venous thromboembolism after surgery for spine metastatic bone lesions: a retrospective study. Clin Orthop Relat Res. 2019;477(7):1674-86.
Cohoon KP, Ransom JE, Leibson CL, Ashrani AA, Petterson TM, Long KH, et al. Direct medical costs attributable to cancer-associated venous thromboembolism: a population-based longitudinal study. Am J Med. 2016;129(9):1000.e15-25.
Rogers SO Jr, Kilaru RK, Hosokawa P, Henderson WG, Zinner MJ, Khuri SF. Multivariable predictors of postoperative venous thromboembolic events after general and vascular surgery: results from the patient safety in surgery study. J Am Coll Surg. 2007;204(6):1211-21.
Caprini JA, Arcelus JI, Reyna JJ. Effective risk stratification of surgical and nonsurgical patients for venous thromboembolic disease. Semin Hematol. 2001;38(2 Suppl 5):12-9.
Barber EL, Clarke-Pearson DL. The limited utility of currently available venous thromboembolism risk assessment tools in gynecological oncology patients. Am J Obstet Gynecol. 2016;215(4):445.e1-9.
Khorana AA, Kuderer NM, Culakova E, Lyman GH, Francis CW. Development and validation of a predictive model for chemotherapy-associated thrombosis. Blood. 2008;111(10):4902-7.
Han SS, Azad TD, Suarez PA, Ratliff JK. A machine learning approach for predictive models of adverse events following spine surgery. Spine J. 2019;19(11):1772-81.
Collins GS, Reitsma JB, Altman DG, Moons KG.Transparent reporting of a multivariable prediction model for individual prognosis or diagnosis (TRIPOD) the TRIPOD statement. Circulation. 2015;131(2):211-9.
Luo W, Phung D, Tran T, Gupta S, Rana S, Karmakar C, et al. Guidelines for developing and reporting machine learning predictive models in biomedical research: a multidisciplinary view. J Med Internet Res. 2016;18(12):e323.
Ikeda T, Miyamoto H, Hashimoto K, Akagi M. Predictable factors of deep venous thrombosis in patients undergoing spine surgery. J Orthop Sci. 2017;22(2):197-200.
Yoshioka K, Murakami H, Demura S, Kato S, Tsuchiya H. Prevalence and risk factors for development of venous thromboembolism after degenerative spinal surgery. Spine (Phila Pa 1976). 2015;40(5):E301-6.
Wei J, Li W, Pei Y, Shen Y, Li J. Clinical analysis of preoperative risk factors for the incidence of deep venous thromboembolism in patients undergoing posterior lumbar interbody fusion. J Orthop Surg Res. 2016;11(1):68.
Yang SD, Liu H, Sun YP, Yang DL, Shen Y, Feng SQ, et al. Prevalence and risk factors of deep vein thrombosis in patients after spine surgery: a retrospective case-cohort study. Sci Rep. 2015;5:11834.
Tominaga H, Setoguchi T, Tanabe F, Kawamura I, Tsuneyoshi Y, Kawabata N, et al. Risk factors for venous thromboembolism after spine surgery. Medicine (Baltimore). 2015;94(5):e466.
Guzman JZ, Latridis JC, Skovrlj B, Cutler HS, Hecht AC, Qureshi SA, et al. Outcomes and complications of diabetes mellitus on patients undergoing degenerative lumbar spine surgery. Spine (Phila Pa 1976). 2014 Sep 1;39(19):1596-604.
Adogwa O, Elsamadicy AA, Sergesketter A, Oyeyemi D, Galan D, Vuong VD, et al. The impact of chronic kidney disease on postoperative outcomes in patients undergoing lumbar decompression and fusion. World Neurosurg. 2018;110:e266-e70.
De la Garza Ramos R, Jain A, Nakhla J, Nasser R, Puvanesarajah V, Hassanzadeh H, et al. Postoperative morbidity and mortality after elective anterior cervical fusion in patients with chronic and end-stage renal disease. World Neurosurg. 2016;95:480-5.
Sebastian AS, Currier BL, Kakar S, Nguyen EC, Wagie AE, Habermann ES, et al. Risk factors for venous thromboembolism following thoracolumbar surgery: analysis of 43,777 patients from the American College of Surgeons National Surgical Quality Improvement Program 2005 to 2012. Global Spine J. 2016;6(8):738-43.
Phan K, Kothari P, Lee NJ, Virk S, Kim JS, Cho SK. Impact of obesity on outcomes in adults undergoing elective posterior cervical fusion. Spine (Phila Pa 1976). 2017;42(4):261-6.
Flippin M, Harris J, Paxton EW, Prentice HA, Fithian DC, Ward SR, et al. Effect of body mass index on patient outcomes of surgical intervention for the lumbar spine. J Spine Surg. 2017;3(3):349-57.
Johnson DJ, Johnson CC, Cohen DB, Wetler JA, Kbaish KM, Frank SM. Thrombotic and infectious morbidity are associated with transfusion in posterior spine fusion. HSS J. 2017;13(2):152-8.
Aoude A, Aldebeyan S, Fortin M, Nooh A, Jarzem P, Ouellet JA, et al. Prevalence and complications of postoperative transfusion for cervical fusion procedures in spine surgery: an analysis of 11,588 patients from the American College of Surgeons National Surgical Quality Improvement Program Database. Asian Spine J. 2017;11(6):880-91.
Aoude A, Nooh A, Fortin M, Aldebeyan S, Jarzem P, Ouellet J, et al. Incidence, predictors, and postoperative complications of blood transfusion in thoracic and lumbar fusion surgery: an analysis of 13,695 patients from the American College of Surgeons National Surgical Quality Improvement Program Database. Global Spine J. 2016;6(8):756-64.
Bekelis K, Desai A, Bakhoum SF, Missios S. A predictive model of complications after spine surgery: the National Surgical Quality Improvement Program (NSQIP) 2005–2010. Spine J. 2014;14(7):1247-55.
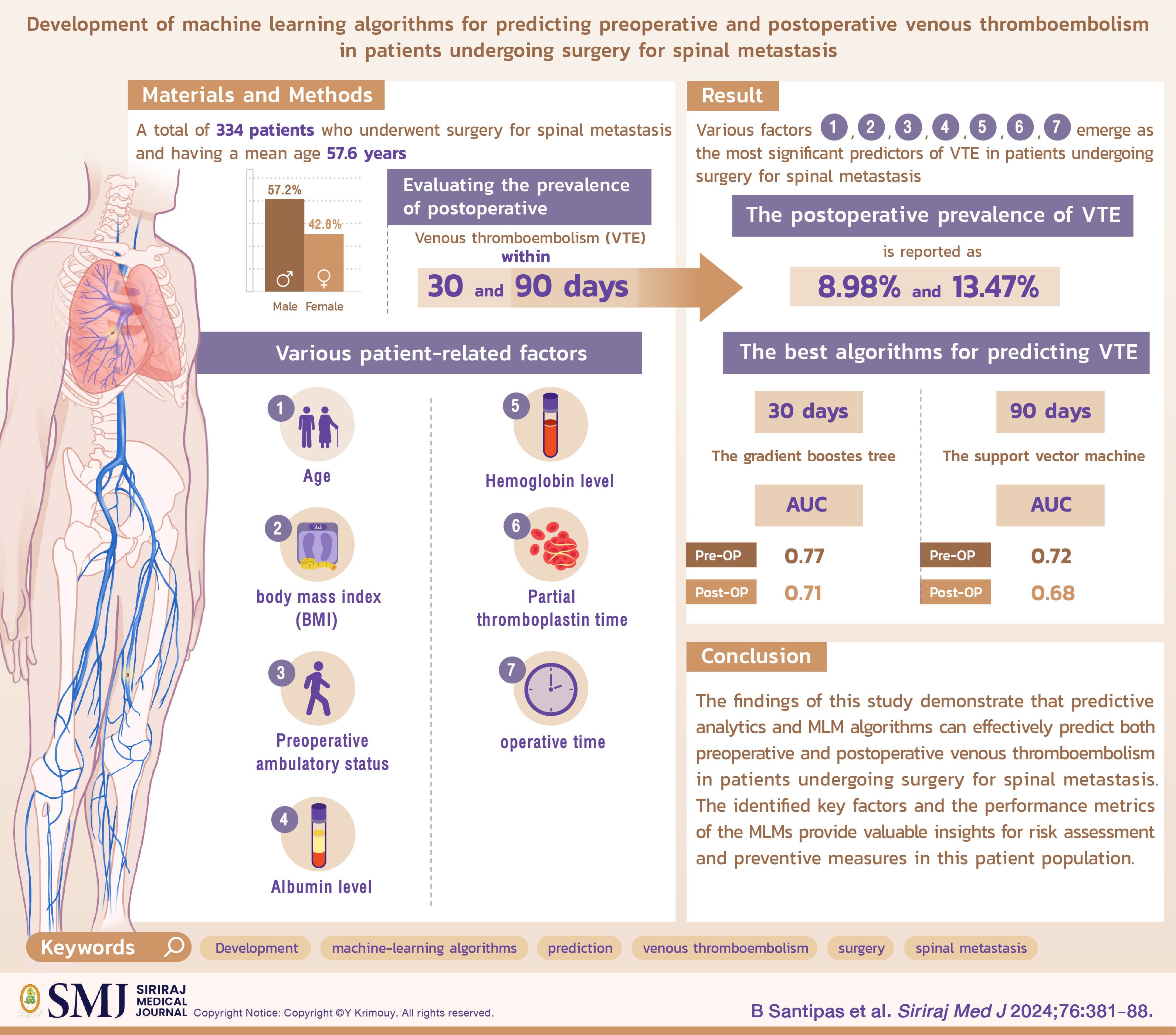
Published
How to Cite
Issue
Section
Categories
License
Copyright (c) 2024 Siriraj Medical Journal

This work is licensed under a Creative Commons Attribution-NonCommercial-NoDerivatives 4.0 International License.
Authors who publish with this journal agree to the following conditions:
Copyright Transfer
In submitting a manuscript, the authors acknowledge that the work will become the copyrighted property of Siriraj Medical Journal upon publication.
License
Articles are licensed under a Creative Commons Attribution-NonCommercial-NoDerivatives 4.0 International License (CC BY-NC-ND 4.0). This license allows for the sharing of the work for non-commercial purposes with proper attribution to the authors and the journal. However, it does not permit modifications or the creation of derivative works.
Sharing and Access
Authors are encouraged to share their article on their personal or institutional websites and through other non-commercial platforms. Doing so can increase readership and citations.