Development and Evaluation of the DMIND Questionnaire: Preparing for AI Integration into an Effective Depression Screening Tool
DOI:
https://doi.org/10.33192/smj.v76i9.269527Keywords:
Depression, Depression Screening, Mental Health, Artificial IntelligenceAbstract
Objective: Thailand’s mental health crisis is exacerbated by high demand and a shortage of mental health professionals. The research objective was to develop and validate the Detection and Monitoring Intelligence Network for Depression (DMIND) questionnaire, designed to be culturally relevant and easily administered in clinical settings. Crafted with expert input, items specifically conducive to artificial intelligence (AI) analysis were selected to facilitate the future development of an AI-assisted depression scoring model. This approach underscores the tool’s dual utility in both human-led and technology-enhanced diagnostics.
Materials and Methods: We enrolled 81 participants from psychiatric and tertiary care hospitals in Bangkok. Participants were assessed using the DMIND questionnaire, followed by the Hamilton Depression Rating Scale (HDRS-17). Statistical analyses included the content validity index (CVI), Cronbach’s alpha, Pearson’s correlation coefficient, Cohen’s kappa, and receiver operating characteristic (ROC) analysis. The Liu method, Youden index, and nearest neighbor method were used to determine the optimal cut-off point
Results: The DMIND questionnaire showed strong validity, with an item-level CVI (I-CVI) and scale-level CVI (S-CVI) exceeding 1.0, indicating strong consensus on its relevance and utility. The tool also demonstrated high internal consistency (Cronbach’s alpha = 0.96). ROC analysis showed an AUC of 0.88, indicating high accuracy in depression screening. An optimal cut-off score of 11.5 was identified, balancing predictive value and sensitivity.
Conclusion: The DMIND questionnaire represents a significant advancement in innovative mental health diagnostics, addressing unmet clinical needs by providing accurate and efficient assessments capable of AI integration for further enhancing mental health service delivery in Thailand.
References
Gotlib IH, Hammen CL. Handbook of depression. 2002. Isacsson G. Suicide prevention--a medical breakthrough? Acta Psychiatr Scand. 2000;102(2):113-7.
Nochaiwong S, Ruengorn C, Thavorn K, Hutton B, Awiphan R, Phosuya C, et al. Global prevalence of mental health issues among the general population during the coronavirus disease-2019 pandemic: a systematic review and meta-analysis. Sci Rep. 2021;11(1):10173.
Prasartpornsirichoke J, Pityaratstian N, Poolvoralaks C, Sirinimnualkul N, Ormtavesub T, Hiranwattana N, et al. The prevalence and economic burden of treatment-resistant depression in Thailand. BMC Public Health. 2023;23(1):1541.
Mitchell AJ, Vaze A, Rao S. Clinical diagnosis of depression in primary care: a meta-analysis. Lancet. 2009;374(9690):609-19.
Carrozzino D, Patierno C, Fava GA, Guidi J. The Hamilton Rating Scales for Depression: A Critical Review of Clinimetric Properties of Different Versions. Psychother Psychosom. 2020;89(3):133-50.
Mathers CD, Loncar D. Projections of global mortality and burden of disease from 2002 to 2030. PLoS Med. 2006;3(11):e442.
Coverage T, Mental Health Hotline 1323.2003. Available from: https://www.thecoverage.info/news/content/4691
Dibeklioglu H, Hammal Z, Cohn JF. Dynamic Multimodal Measurement of Depression Severity Using Deep Autoencoding. IEEE J Biomed Health Inform. 2018;22(2):525-36.
Jiang Z, Seyedi S, Griner E, Abbasi A, Rad AB, Kwon H, et al. Multimodal Mental Health Digital Biomarker Analysis from Remote Interviews using Facial, Vocal, Linguistic, and Cardiovascular Patterns. IEEE J Biomed Health Inform. 2024;28(3):1680-91.
Lee EE, Torous J, De Choudhury M, Depp CA, Graham SA, Kim HC, et al. Artificial Intelligence for Mental Health Care: Clinical Applications, Barriers, Facilitators, and Artificial Wisdom. Biol Psychiatry Cogn Neurosci Neuroimaging. 2021;6(9):856-64.
Williams SZ, Chung GS, Muennig PA. Undiagnosed depression: A community diagnosis. SSM Popul Health. 2017;3:633-8.
Rajpurkar P, Chen E, Banerjee O, Topol EJ. AI in health and medicine. Nat Med. 2022;28(1):31-38.
Shin D, Cho WI, Park CHK, Rhee SJ, Kim MJ, Lee H, et al. Detection of Minor and Major Depression through Voice as a Biomarker Using Machine Learning. J Clin Med. 2021;10(14):3046.
Spitzer RL, Kroenke K, Williams JB. Validation and utility of a self-report version of PRIME-MD: the PHQ primary care study. Primary Care Evaluation of Mental Disorders. Patient Health Questionnaire. JAMA. 1999;282(18):1737-44.
Karimollah HT. Sample size estimation in diagnostic test studies of biomedical informatics. J Biomed Inform. 2014;48:193-204.
Hamilton M. A rating scale for depression. J Neurol Neurosurg Psychiatry. 1960;23:56-62.
Lotrakul M, Sukanich P, Sukying C. The Reliability and Validity of Thai version of Hamilton Rating Scale for Depression. Journal of the Psychiatrist Association of Thailand. 1996;41(4):235-46.
Kroenke K, Spitzer RL, Williams JB. The PHQ-9: validity of a brief depression severity measure. J Gen Intern Med. 2001;16(9):606-13.
Sumrithe S, Saipanish R. Reliability and validity of the Thai version of the PHQ-9. BMC Psychiatry. 2008;8:46.
Gilbody S, Richards D, Brealey S, et al. Screening for depression in medical settings with the Patient Health Questionnaire (PHQ): a diagnostic meta-analysis. J Gen Intern Med. 2007;22(11):1596-602.
Liu X. Classification accuracy and cut point selection. Stat Med. 2012;31(23):2676-86.
Youden WJ. Index for rating diagnostic tests. Cancer. 1950;3(1):32-35.
Beck AT, Ward CH, Mendelson M, Mock J, Erbaugh J. An inventory for measuring depression. Arch Gen Psychiatry.1961;4(6):561-71.
Radloff LS. The CES-D Scale: A self-report report depression scale for research in the general population. Applied Psychological Measurement. 1977;1(3):385-401.
Montgomery SA, Åsberg M. A new depression scale designed to be sensitive to change. Br J Psychiatry.1979;134:382-9.
Torres SC, Olofsdotter S, Vadlin S, Ramklint M, Nilsson KW, Sonnby K. Diagnostic accuracy of the Montgomery–Åsberg Depression Rating Scale parent report among adolescent psychiatric outpatients. Nord J Psychiatry. 2017;72(3):184-90.
Spitzer RL, Kroenke K, Williams JBW. Patient Health Questionnaire Primary Care Study Group. Validation and utility of a self-report version of PRIME-MD: The PHQ primary care study. JAMA. 1999;282(18):1737-44.
Yesavage JA, Brink TL, Rose TL, Lum O, Huang V, Adey M, et al. Development and validation of a Geriatric Depression Screening Scale: A preliminary report. J Psychiatr Res. 1982;17(1):37-49.
Uddin MZ, Dysthe KK, Folstad A, Brandtzaeg PB. Deep learning for prediction of depressive symptoms in a large textual dataset. Neural Computing and Applications, 2022;34:721-44.
Guohou S, Lina Z, Dongsong Z. What reveals about depression level? The role of multimodal features at the level of interview questions. Information & Management. 2020;57(7):103349.
Amanat A, Rizwan M, Javed AR, Abdelhaq M, Alsaqour R, Pandya S, et al. Deep learning for depression detection from textual data. Electronics. 2022;11(5):676.
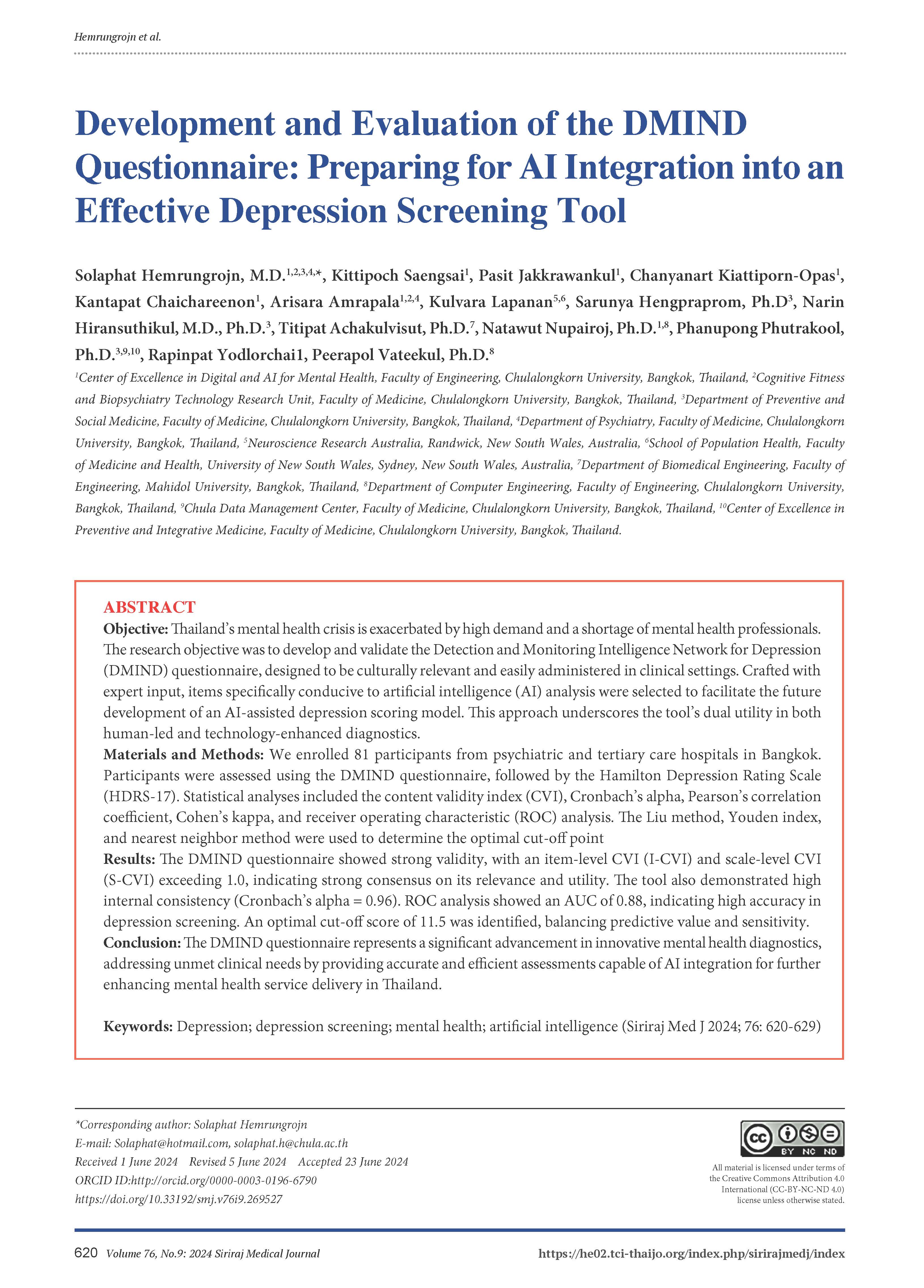
Published
How to Cite
License
Copyright (c) 2024 Siriraj Medical Journal

This work is licensed under a Creative Commons Attribution-NonCommercial-NoDerivatives 4.0 International License.
Authors who publish with this journal agree to the following conditions:
Copyright Transfer
In submitting a manuscript, the authors acknowledge that the work will become the copyrighted property of Siriraj Medical Journal upon publication.
License
Articles are licensed under a Creative Commons Attribution-NonCommercial-NoDerivatives 4.0 International License (CC BY-NC-ND 4.0). This license allows for the sharing of the work for non-commercial purposes with proper attribution to the authors and the journal. However, it does not permit modifications or the creation of derivative works.
Sharing and Access
Authors are encouraged to share their article on their personal or institutional websites and through other non-commercial platforms. Doing so can increase readership and citations.