การเตือนภัยการระบาดล่วงหน้าของโรคไข้เลือดออกในประเทศไทยโดยใช้ปัจจัยด้านกีฏวิทยา ระบาดวิทยา และอุตุนิยมวิทยา โดยการวิเคราะห์ด้วยสถิติขั้นสูงและระบบสารสนเทศภูมิศาสตร์
คำสำคัญ:
โรคไข้เลือดออก, กีฏวิทยา, ระบาดวิทยา, อุตุนิยมวิทยา, ระบบสารสนเทศภูมิศาสตร์บทคัดย่อ
การระบาดของโรคไข้เลือดออกมีหลายปัจจัยที่เกี่ยวข้อง โดยที่โรคนี้ยังเป็นปัญหาสาธารณสุข การเตือนภัยล่วงหน้าเป็นมาตรการหนึ่งสำหรับการควบคุมโรค งานวิจัยนี้ใช้ปัจจัยด้านกีฏวิทยา ระบาดวิทยา และอุตุนิยมวิทยา นำมาวิเคราะห์ด้วยสถิติขั้นสูงและระบบสารสนเทศภูมิศาสตร์เพื่อเตือนภัยโรคไข้เลือดออก ในการศึกษาเลือกจังหวัดตัวแทนภาคละ 8 จังหวัด รวม 32 จังหวัด และใช้ข้อมูลระหว่างเดือนมกราคม-พฤษภาคมของปี พ.ศ. 2555-2562 โดยนำปัจจัยผู้ป่วยโรคไข้เลือดออกต่อแสนประชากร (X1) ค่าเฉลี่ยอุณหภูมิ (X2) ค่าเฉลี่ยความชื้นสัมพัทธ์ (X3) ค่าเฉลี่ยปริมาณนํ้าฝน (X4) และค่าเฉลี่ยจำนวนไข่ต่อกับดักไข่ยุงลาย (X5) มาวิเคราะห์หาแบบจำลองตามช่วงที่กำหนดสำหรับแต่ละภาค ผลการคาดการณ์ผู้ป่วยโรคไข้เลือดออกต่อแสนประชากร (y) โดยวิธีวิเคราะห์ถดถอยหลายตัวแปร มีค่า R2 อยู่ในช่วง 0.616-0.647 และการคาดการณ์โอกาสการระบาดโรคไข้เลือดออก [logist(y)] ด้วยวิธีวิเคราะห์ถดถอยโลจิสติคพหุกลุ่ม มีค่า Overall Correct อยู่ในช่วง 73.750-79.200 เมื่อนำมาวิเคราะห์ด้วยวิธีการซ้อนทับข้อมูลตามเงื่อนไขที่กำหนดของ 77 จังหวัด สร้างเป็นแผนที่ระบบสารสนเทศภูมิศาสตร์ เปรียบเทียบผลการคาดการณ์ที่ได้กับค่าจริง พบร้อยละความแม่นยำของการคาดการณ์โรคไข้เลือดออกในปี พ.ศ. 2559, 2560 และ 2562 มีค่าอยู่ในช่วง 67.408-84.793 ดังนั้นแบบจำลองและวิธีการที่ได้สามารถนำไปใช้คาดการณ์การระบาดของโรคในแต่ละจังหวัดของปีที่ต้องการ เพียงใส่ข้อมูลปัจจัยช่วงต้นปี ก็จะทราบแนวโน้มการระบาดล่วงหน้า 2-3 เดือน มีประโยชน์ในการช่วยเตือนภัย ทำให้มีเวลาเตรียมการและดำเนินการควบคุมยุงลาย รวมทั้งช่วยในการวางแผนการควบคุมโรคไข้เลือดออกล่วงหน้าได้อย่างมีประสิทธิภาพในแต่ละจังหวัดของประเทศไทย
References
Hermann LL, Gupta SB, Manoff SB, Kalayanarooj S, Gibbons RV, Coller BAG. Advances in the understanding, management, and prevention of dengue. J Clin Virol 2015; 64: 153-9.
Wilder-Smith A, Ooi EE, Horstick O, Wills B. Dengue. Lancet 2019; 393(10169): 350-63.
Limkittikul K, Brett J, L’Azou M. Epidemiological trends of dengue disease in Thailand (2000-2011): a systematic literature review. PLoS Negl Trop Dis 2014; 8(11): e3241. (10 pages).
Gratz NG. Lessons of Aedes aegypti control in Thailand. Med Vet Entomol 1993; 7(1): 1-10.
Racloz V, Ramsey R, Tong S, Hu W. Surveillance of dengue fever virus: a review of epidemiological models and early warning systems. PLoS Negl Trop Dis 2012; 6(5): e1648. (9 pages).
Sivaprasad A, Beevi NS, Manojkumar TK. Dengue and Early Warning Systems: a review based on Social Network Analysis. Procedia Comput Sci 2020; 171: 253-62.
Myers MF, Rogers DJ, Cox J, Flahault A, Hay SI. Forecasting disease risk for increased epidemic preparedness in public health. Adv Parasitol 2000; 47: 309-30.
Promprou S, Jaroensutasinee M, Jaroensutasinee K. Forecasting dengue haemorrhagic fever cases in Southern Thailand using ARIMA Models. Dengue Bull 2006; 30: 99-106.
Wongkoon S, Jaroensutasinee M, Jaroensutasinee K. Development of temporal modeling for prediction of dengue infection in Northeastern Thailand. Asia Pac J Trop Med 2012; 5(3): 249-52.
สำนักโรคติดต่อนำโดยแมลง กรมควบคุมโรค. คู่มือวิชาการโรคติดเชื้อเดงกีและโรคไข้เลือดออกเดงกีด้านการแพทย์และสาธารณสุข. [ออนไลน์]. 2558; [สืบค้น 6 มี.ค. 2564]; [152หน้า]. เข้าถึงได้ที่: URL: https://www.pidst.or.th/A434.html?action=download&file=481_คู่มือวิชาการโรคไข้เลือดออกปี2558_-%20%20FINAL.pdf.
Morrison AC, Zielinski-Gutierrez E, Scott TW, Rosenberg R. Defi ning challenges and proposing solutions for control of the virus vector Aedes aegypti. PloS Med 2008; 5(3): e68. (5 pages).
Chien LC, Yu HL. Impact of meteorological factors on the spatiotemporal patterns of dengue fever incidence. Environ Int 2014; 73: 46-56.
Descloux E, Mangeas M, Menkes CE, Lengaigne M, Leroy A, Tehei T, et al. Climate-based models for understanding and forecasting dengue epidemics. PLoS Negl Trop Dis 2012; 6(2): e1470. (19 pages).
Campbell KM, Lin CD, Iamsirithaworn S, Scott TW. The Complex relationship between weather and dengue virus transmission in Thailand. Am J Trop Med Hyg 2013; 89(6): 1066-80.
World Health Organization. Public health mapping and GIS for global health security: a WHO strategic and operational framework. [online]. 2006; [cited 2021 Mar 19]; [22 screens] Available from: URL: https://apps.who.int/iris/bitstream/handle/10665/69715/WHO_CDS_GIS_2006.1_eng.pdf
Louis VR, Phalkey R, Horstick O, Ratanawong P, Wilder-Smith A, Tozan Y, et al. Modeling tools for dengue risk mapping a systematic review. Int J Health Geogr 2014; 13: 50. 1(15 pages).
จิตติ จันทร์แสง, จิรัติวัล เครือศิลป์, สุรภี อนันตปรีชา, อุรุญากร จันทร์แสง, อุษาวดี ถาวระ, รุ่งเรือง กิจผาติ และคณะ. ระบบสารสนเทศภูมิศาสตร์-โรคไข้เลือดออกสำหรับการหาพื้นที่เสี่ยงการเกิดโรคระดับอำเภอโดยใช้ข้อมูลระบาดวิทยาและข้อมูลภาพดาวเทียม. ใน: การประชุมวิชาการวิทยาศาสตร์การแพทย์ ครั้งที่ 15. วันที่ 27-29 สิงหาคม 2550. นนทบุรี: กรมวิทยาศาสตร์การแพทย์ กระทรวงสาธารณสุข; 2550. หน้า 39.
จิตติ จันทร์แสง, บุษยา ธูปพุดซา, สุรภี อนันตปรีชา, อุรุญากร จันทร์แสง, รุ่งเรือง กิจผาติ, ปฐม สวรรค์ปัญญาเลิศ. การพยากรณ์โรคไข้เลือดออกเชิงพื้นที่ด้วยระบบสารสนเทศภูมิศาสตร์ (GIS) และข้อมูลระบาดวิทยาเชิงคุณภาพ. ใน: การประชุมวิชาการวิทยาศาสตร์การแพทย์ ครั้งที่ 16. วันที่ 12-13 มิถุนายน 2551. นนทบุรี: กรมวิทยาศาสตร์การแพทย์ กระทรวงสาธารณสุข; 2551. หน้า 23.
จิตติ จันทร์แสง, อุรุญากร จันทร์แสง, สมชาย แสงกิจพร, นิพนธ์ โพธิ์พัฒนชัย. ระบบสารสนเทศภูมิศาสตร์-โรคไข้เลือดออกเพื่อการพยากรณ์โอกาสการเกิดการระบาดและอัตราผู้ป่วยระดับจังหวัด. ใน: การประชุมวิชาการวิทยาศาสตร์การแพทย์ ครั้งที่ 21. วันที่ 16-18 มิถุนายน 2556. นนทบุรี: กรมวิทยาศาสตร์การแพทย์ กระทรวงสาธารณสุข; 2556. หน้า 58.
สำนักระบาดวิทยา กรมควบคุมโรค. จำนวนผู้ป่วย-เสียชีวิตรายเดือน แยกรายจังหวัด, จำนวนและอัตราป่วย-ตายต่อแสนประชากร แยกรายจังหวัด. [ออนไลน์]. 2563; [สืบค้น 25 มีนาคม 2564]; [14 หน้า]. เข้าถึงได้ที่: URL: http://www.boe.moph.go.th/boedb/surdata/disease.php?dcontent=old&ds=262766.
กรมอุตุนิยมวิทยา. การสรุปลักษณะอากาศรายปี. [ออนไลน์]. 2558; [สืบค้น 25 มีนาคม 2564]. เข้าถึงได้ที่: URL: http://climate.tmd.go.th/content/category/17.
จิตติ จันทร์แสง, จริยา ครุธบุตร์, ธัณญภักษณ์ มากรื่น, วรรณิศา สืบสอาด, เอกรัฐ เด่นชลชัย, สุวรรณภา นิลพนมชัย, และคณะ. พื้นที่เสี่ยงโรคไข้เลือดออก: วิเคราะห์ผลการสำรวจกับดักไข่ยุงลายและข้อมูลผู้ป่วยโรคไข้เลือดออกของประเทศไทยด้วยระบบสารสนเทศภูมิศาสตร์. ว กรมวิทย พ 2564; 63(1): 78-91.
Katz MH. Multivariable analysis: a practical guide for clinicians. Cambridge: Cambridge University Press; 1999.
สุชาติ ประสิทธิ์รัฐสินธุ์. การใช้สถิติในงานวิจัยอย่างถูกต้องและได้มาตรฐานสากล. พิมพ์ครั้งที่ 6. กรุงเทพฯ: สามลดา; 2556.
Norusis, MJ. SPSS/PC+. Chicago: SPSS Inc.; 1988.
จิตติ จันทร์แสง, เบญญาภา ธูปพุดซา, อารีรัตน์ สง่าแสง, อุรุญากร จันทร์แสง, สมชาย แสงกิจพร. แผนที่ GIS เพื่อการประมาณค่าเชิงพื้นที่ของประเทศ จากผลการตรวจทางห้องปฏิบัติการด้านวิทยาศาสตร์การแพทย์: กรณีศึกษาการตรวจไวรัสเดงกีและประชากรยุงลายพาหะนำโรคไข้เลือดออก. ใน: การประชุมวิชาการวิทยาศาสตร์การแพทย์ ครั้งที่ 22. วันที่ 30 มิถุนายน – 2 กรกฎาคม 2557. นนทบุรี: กรมวิทยาศาสตร์การแพทย์ กระทรวงสาธารณสุข; 2557. หน้า 68-69.
ESRI. ArcGIS 9: using ArcGIS desktop. New York: Esri Press; 2006.
จิตติ จันทร์แสง, ประคอง พันธ์อุไร, อุษาวดี ถาวระ, อุรุญากร จันทร์แสง, อภิวัฏ ธวัชสิน, สุพล เป้าศรีวงษ์. รูปแบบสำหรับการพยากรณ์จำนวนผู้ป่วยโรคไข้เลือดออกในเขตภาคตะวันออกเฉียงเหนือ. วารสารโรคติดต่อ 2540; 23(1): 72-8.
World Health Organization. Early warning and response system (EWARS) for dengue outbreaks: operational guide using the web-based dashboard. [online]. 2018; [cited 2021 Mar 22]; [52 screens] Available from: URL: https://apps.who.int/iris/bitstream/handle/10665/274318/9789241514354-eng.pdf?sequence=1&isAllowed=y.
คณะกรรมการวางแผนพัฒนาการสาธารณสุข. แผนพัฒนาการสาธารณสุขตามแผนพัฒนาการเศรษฐกิจและสังคมแห่งชาติ ฉบับที่ 7 (พ.ศ. 2535-2539). กรุงเทพฯ: โรงพิมพ์องค์การสงเคราะห์ทหารผ่านศึก; 2535.
จิตติ จันทร์แสง. การประยุกต์ใช้ระบบสารสนเทศทางภูมิศาสตร์และการสำรวจข้อมูลระยะไกลเพื่อการประเมินความเสี่ยงต่อไข้เลือดออกในประเทศไทย. [วิทยานิพนธ์]. ภาควิชาชีววิทยา, บัณฑิตวิทยาลัย. นครปฐม: มหาวิทยาลัยมหิดล; 2548.
จิรัติวัล เครือศิลป์. ระบบสารสนเทศทางภูมิศาสตร์เพื่อกำหนดพื้นที่เสี่ยงต่อการเกิดโรคไข้เลือดออกในจังหวัดนครราชสีมา. [วิทยานิพนธ์]. ภาควิชาภูมิศาสตร์, คณะอักษรศาสตร์. กรุงเทพฯ: จุฬาลงกรณ์มหาวิทยาลัย; 2549.
สมหวัง คุรุศาสตรา. การประเมินความเสี่ยงการระบาดของโรคไข้เลือดออกโดยใช้การวิเคราะห์เชิงพื้นที่ในจังหวัดฉะเชิงเทรา. [วิทยานิพนธ์]. ภาควิชานิเวศวิทยาอุตสาหกรรมและสิ่งแวดล้อม, บัณฑิตวิทยาลัย. นครปฐม: มหาวิทยาลัยมหิดล; 2553.
Ninphanomchai S, Chansang C, Hii YL, Rocklöv J, Kittayapong P. Predictiveness of disease risk in a global outreach tourist setting in Thailand using meteorological data and vector-borne disease incidences. Int J Environ Res Public Health 2014; 11(10): 10694-709.
QGIS Develop Team. QGIS: a free and open source geographic information system. [online]. 2021; [cited 2021 Mar 29]; [3 screens]. Available from: URL: https://qgis.org/en/site.
MapWindow Open Source Team. MapWindow GIS. [online]. 2021; [cited 2021 Mar 29]; [8 screens] Available from: URL: https://www.mapwindow.org.
จิตติ จันท์แสง. การประยุกต์ใช้ GIS, GPS, และ RS สำหรับการทำแผนที่และงานวิจัยด้านสาธารณสุข [วิดีโอออนไลน์]. 2563; [สืบค้น 31 มี.ค. 2564]. เข้าถึงได้ที่: URL: https://www.youtube.com/watch?v=xxGxU0bovL8.
สำนักโรคติดต่อนำโดยแมลง กรมควบคุมโรค. รายงานพยากรณ์โรคไข้เลือดออก ปี 2560. [ออนไลน์]. 2560; [สืบค้น 13 มี.ค. 2564]; [43 หน้า]. เข้าถึงได้ที่: URL: https://ddc.moph.go.th/uploads/publish/1026520200625043647.pdf.
สำนักโรคติดต่อนำโดยแมลง กรมควบคุมโรค. รายงานพยากรณ์โรคไข้เลือดออก ปี 2562. [ออนไลน์]. 2562; [สืบค้น 13 มี.ค. 2564]; [53 หน้า]. เข้าถึงได้ที่: URL: https://ddc.moph.go.th/uploads/ckeditor/6f4922f45568161a8cdf4ad2299f6d23/files/Dangue/Prophecy/2562.pdf.
จิตติ จันท์แสง และคณะ. สถานการณ์ยุงลายและพื้นที่เสี่ยงโรคไข้เลือดออก. [ออนไลน์]. 2559; [สืบค้น 30 มี.ค. 2564]; [2 หน้า]. เข้าถึงได้ที่: URL: http://nih.dmsc.moph.go.th/login/showimgdetil.php?id=1110.
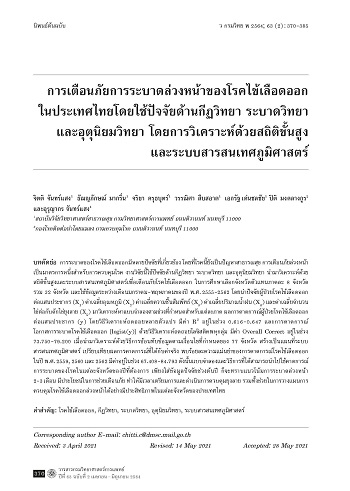
Downloads
เผยแพร่แล้ว
How to Cite
ฉบับ
บท
License
Copyright (c) 2021 วารสารกรมวิทยาศาสตร์การแพทย์

This work is licensed under a Creative Commons Attribution-NonCommercial-NoDerivatives 4.0 International License.